Diagnosing Adolescent Substance Use and Co‐Occurring Disorders Using the Global Appraisal of Individual Needs Quick Version‐4
Abstract
Objective
Methods
Results
Conclusions
Trial registration
HIGHLIGHTS
METHODS
Participants & Procedures
Measures
Data Analysis
RESULTS
Sample Characteristics
Characteristics | Adolescents (12–17) | Adults (18 +) | OR (95% CI) |
---|---|---|---|
N = 101,897 | N = 204,711 | ||
Age‐ mean (SD) | 15.6 (1.25) | 34.2 (11.67) | n/a |
Male (%) | 71.5% | 61.7% | 1.56 (1.53–1.58) |
Race/ethnicity | |||
Non‐Hispanic African American (%) | 24.0% | 16.5% | 1.6 (1.58–1.63) |
Non‐Hispanic Caucasian (%) | 47.8% | 65.7% | 0.48 (0.47–0.49) |
Hispanic (%) | 26.7% | 14.7% | 2.12 (2.08–2.16) |
Mixed/other (%) | 13.1% | 7.3% | 1.91 (1.86–1.95) |
Assessment setting | |||
Legal system | 9.3% | 15.8% | 0.55 (0.54–0.56) |
Treatment | 53.7% | 70.8% | 0.48 (0.47–0.49) |
Home/office | 19.1% | 3.0% | 7.7 (7.47–7.93) |
Other | 10.5% | 3.9% | 2.87 (2.79–2.96) |
Unknown | 7.3% | 6.5% | 1.13 (1.1–1.17) |
Past 90‐day use of alcohol (%) | 50.3% | 49.3% | 1.04 (1.03–1.06) |
Past 90‐day use of cannabis (%) | 73.5% | 35.0% | 5.14 (5.05–5.23) |
Past 90‐day use of stimulants (%) | 12.4% | 30.7% | 0.32 (0.31–0.33) |
Past 90‐day use of opioids (%) | 10.1% | 25.4% | 0.33 (0.32–0.34) |
Past 90‐day use of other (%) | 16.7% | 14.2% | 1.21 (1.18–1.23) |
Any past year needle use (%) | 1.7% | 20.5% | 0.07 (0.06–0.07) |
Current involvement in legal system (%) | 67.8% | 67.9% | 0.99 (0.98–1.01) |
Any prior diagnoses (%) | 37.3% | 51.3% | 0.57 (0.56–0.58) |
Alcohol or drug use disorders (%) | 2.7% | 10.2% | 0.24 (0.23–0.25) |
Attention‐deficit/hyperactivity disorder (%) | 21.5% | 13.8% | 1.71 (1.67–1.74) |
Antisocial personality disorder (%) | 0.2% | 1.2% | 0.18 (0.16–0.21) |
Anxiety or phobia disorder (%) | 6.8% | 20.6% | 0.28 (0.27–0.29) |
Borderline personality disorder (%) | 0.5% | 2.5% | 0.18 (0.16–0.2) |
Conduct disorder (%) | 1.0% | 0.6% | 1.74 (1.59–1.91) |
Major depression disorder (%) | 6.4% | 16.0% | 0.36 (0.35–0.37) |
Other depression, bipolar or mood disorder (%) | 12.1% | 24.7% | 0.42 (0.41–0.43) |
Intellectual disabilities (%) | 0.2% | 0.4% | 0.5 (0.42–0.59) |
Oppositional defiant disorder (%) | 2.1% | 0.6% | 3.83 (3.56–4.13) |
Pathological gambling (%) | 0.0% | 0.0% | 0.24 (0.12–0.46) |
Post or acute traumatic stress disorder (%) | 2.6% | 9.5% | 0.25 (0.24–0.26) |
Somatoform, pain, sleep, eating or body disorder (%) | 0.7% | 1.1% | 0.65 (0.59–0.71) |
Other cognitive disorder (%) | 0.0% | 0.1% | 0.34 (0.24–0.48) |
Other mental breakdown, nerves, or stress (%) | 0.2% | 0.7% | 0.28 (0.24–0.33) |
Other personality disorder (%) | 0.5% | 2.4% | 0.22 (0.2–0.25) |
Other schizophrenia or psychotic disorder (%) | 0.4% | 3.6% | 0.12 (0.11–0.13) |
Other (%) | 2.1% | 2.3% | 0.94 (0.89–0.99) |
Prevalence
Past year mental health diagnosis | Prevalence GAIN Q‐4 | Prevalence GAIN‐I | N of yes | Kappa | Sensitivity | Specificity | Percent agreement |
---|---|---|---|---|---|---|---|
Substance use disorder probable diagnosis | 58% | 61% | 57,945 | 0.80 | 89% | 93% | 90% |
Cannabis | 54% | 50% | 53,887 | 0.71 | 91% | 80% | 85% |
Alcohol | 46% | 17% | 45,984 | 0.33 | 92% | 63% | 68% |
Stimulant | 17% | 7% | 16,768 | 0.48 | 93% | 89% | 89% |
Opioid | 15% | 4% | 15,126 | 0.34 | 97% | 88% | 88% |
Other drug | 22% | 5% | 22,146 | 0.31 | 95% | 82% | 82% |
Internalizing mental health probable diagnosis | 38% | 37% | 37,853 | 0.74 | 85% | 89% | 88% |
Mood | 27% | 30% | 26,789 | 0.68 | 73% | 93% | 87% |
Anxiety | 26% | 10% | 26,235 | 0.50 | 99% | 83% | 85% |
Trauma | 25% | 22% | 24,926 | 0.76 | 85% | 93% | 91% |
Suicide | 11% | 12% | 11,285 | 0.97 | 95% | 100% | 99% |
Possible psychosis | 7% | 7% | 6640 | 1.00 | 100% | 100% | 100% |
Externalizing mental health probable diagnosis | 55% | 51% | 54,388 | 0.72 | 90% | 82% | 86% |
Conduct | 45% | 42% | 45,119 | 0.68 | 85% | 84% | 84% |
ADHD (Attention‐ deficit/hyperacticity disorder) | 52% | 32% | 51,781 | 0.59 | 96% | 72% | 80% |
Gambling | 1% | 1% | 1160 | 0.41 | 73% | 99% | 99% |
3+ of above problems | 69% | 53% | 70,560 | 0.59 | 97% | 62% | 80% |
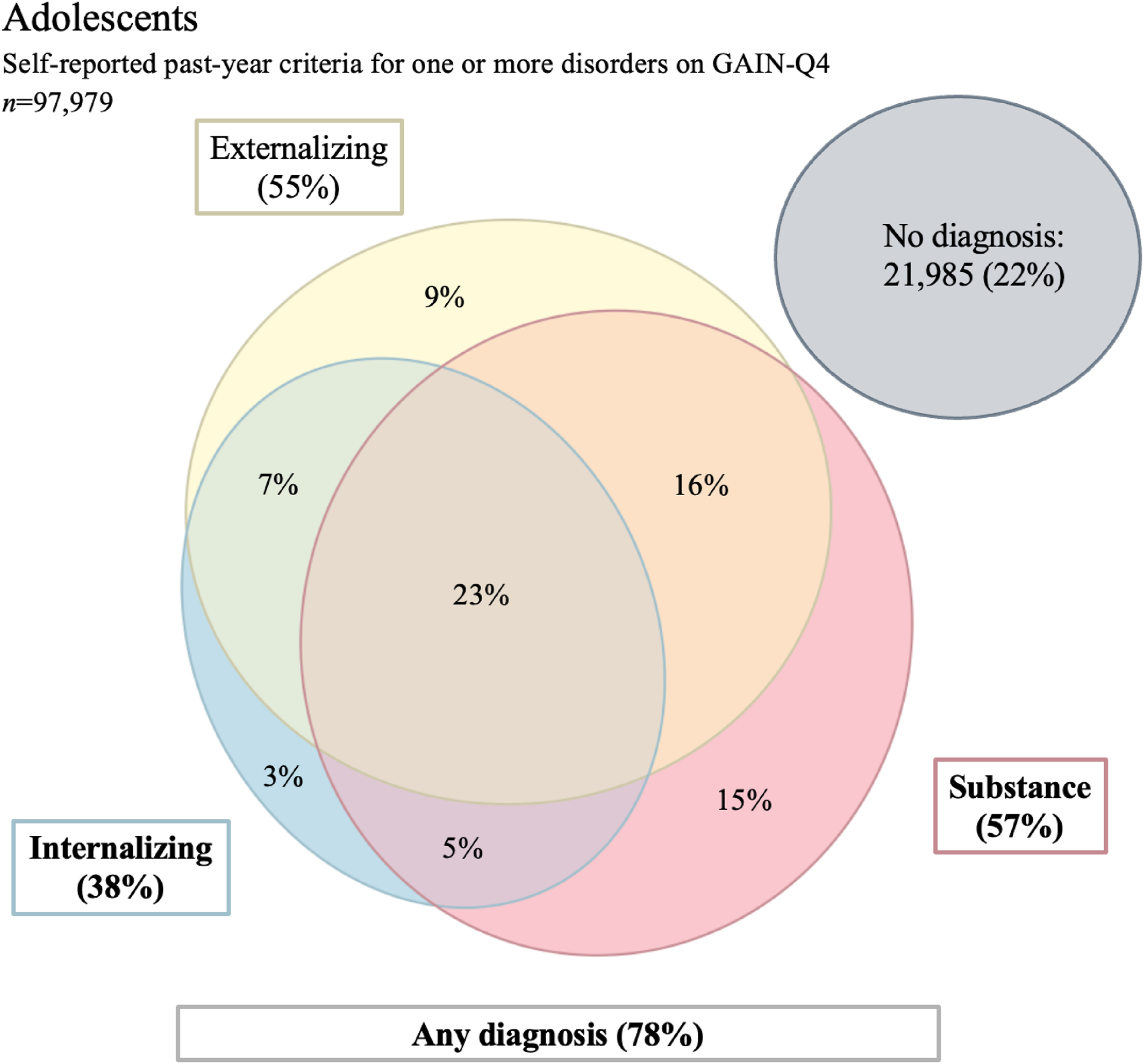
Sensitivity and Specificity
Agreement
DISCUSSION
Footnotes
Supplementary Material
- Download
- 18.12 KB
REFERENCES
Information & Authors
Information
Published In
History
Authors
Funding Information
Metrics & Citations
Metrics
Citations
Export Citations
If you have the appropriate software installed, you can download article citation data to the citation manager of your choice. Simply select your manager software from the list below and click Download.
For more information or tips please see 'Downloading to a citation manager' in the Help menu.
View Options
View options
PDF/EPUB
View PDF/EPUBLogin options
Already a subscriber? Access your subscription through your login credentials or your institution for full access to this article.
Personal login Institutional Login Open Athens loginNot a subscriber?
PsychiatryOnline subscription options offer access to the DSM-5-TR® library, books, journals, CME, and patient resources. This all-in-one virtual library provides psychiatrists and mental health professionals with key resources for diagnosis, treatment, research, and professional development.
Need more help? PsychiatryOnline Customer Service may be reached by emailing [email protected] or by calling 800-368-5777 (in the U.S.) or 703-907-7322 (outside the U.S.).