Prevalence and Penetrance of Rare Pathogenic Variants in Neurodevelopmental Psychiatric Genes in a Health Care System Population
Abstract
Objective:
Methods:
Results:
Conclusions:
Methods
Participants
Exome Sequencing and Variant Calling
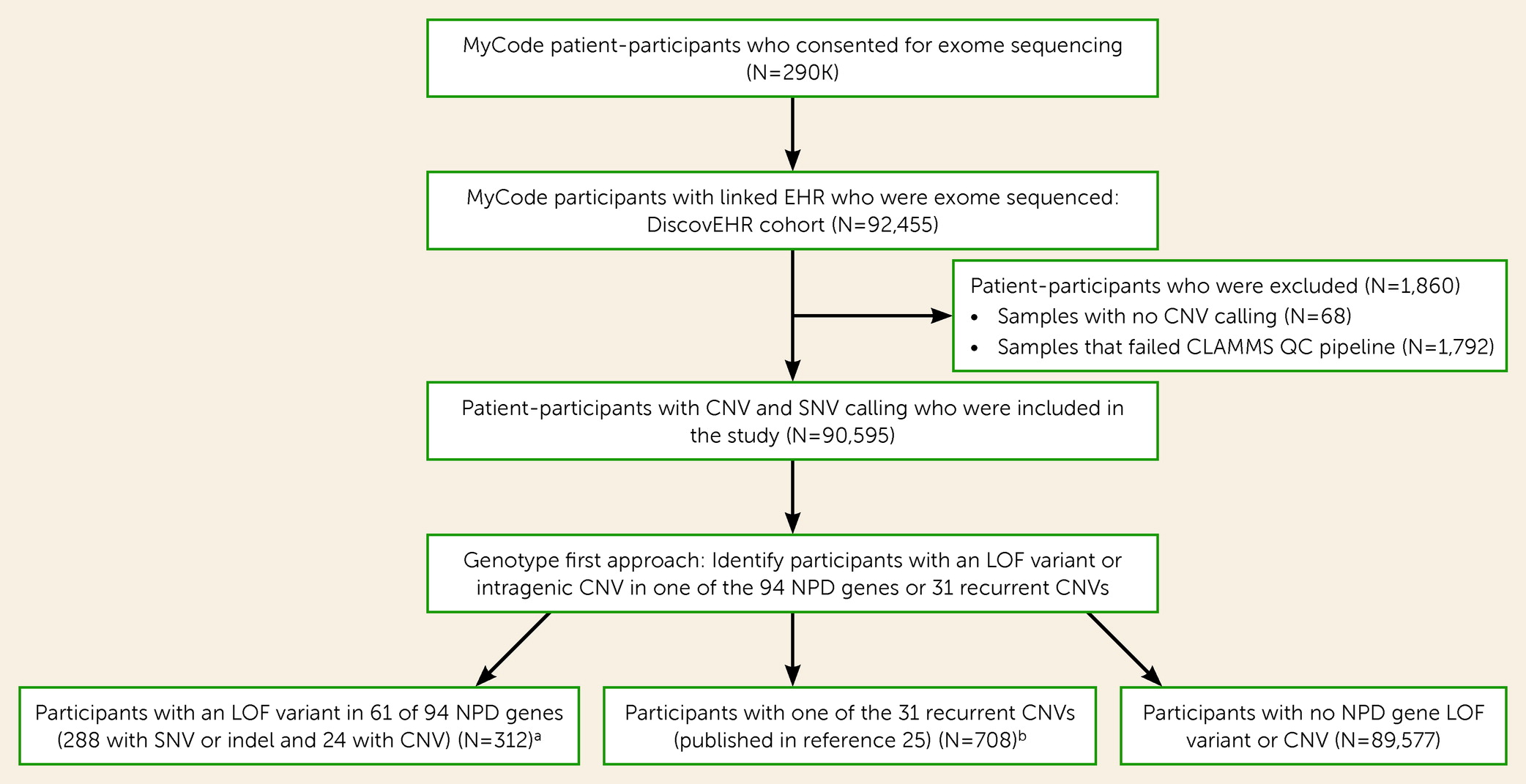
Evidence-Based Approach to NPD Gene Selection
LOF Variant Frequency in NPD Genes From the DiscovEHR and gnomAD Non-Finnish European (NFE) Populations
NPD Diagnosis Extraction
Analysis of Prevalence and Penetrance of Pathogenic Variants
Statistical Analysis
Results
Prevalence of NPD-Related Single-Gene Variants
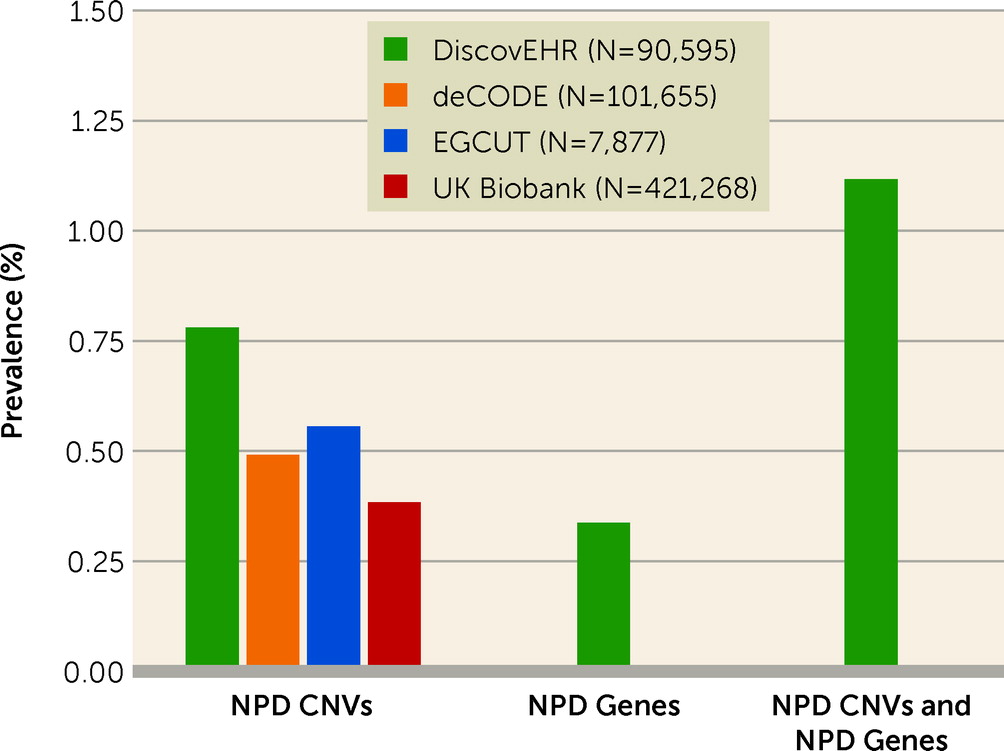
Estimated Penetrance of NPD Gene Variants
Associations Between Rare Genomic Variants and NPD Diagnosis
Disorder | Odds Ratio | 95% CI | Corrected p | Uncorrected p | Cases With a Variant (N) | Total Cases (N) | Controls With a Variant (N) | Total Controls (N) |
---|---|---|---|---|---|---|---|---|
Intellectual disability | 8.73 | 4.83, 14.72 | 1.91×10–8 | 1.36×10–9 | 15 | 423 | 260 | 81,304 |
ASD | 4.56 | 1.58, 11.35 | 0.10 | 6.85×10–3 | 6 | 229 | 269 | 81,498 |
ADHD | 2.89 | 1.83, 4.40 | 2.57×10–4 | 1.84×10–5 | 27 | 2,397 | 248 | 79,330 |
Epilepsy | 2.39 | 1.56, 3.51 | 2.11×10–3 | 1.51×10–4 | 26 | 3,311 | 249 | 78,416 |
Schizophrenia and related disorders | 2.88 | 1.75, 4.45 | 1.47×10–3 | 1.05×10–4 | 19 | 2,234 | 256 | 79,493 |
Bipolar disorder | 2.32 | 1.54, 3.39 | 2.04×10–3 | 1.45×10–4 | 29 | 3,636 | 246 | 78,091 |
Communication disorder | 3.47 | 1.86, 5.97 | 3.92×10–3 | 2.80×10–4 | 13 | 901 | 262 | 80,826 |
Specific learning disorder | 5.52 | 2.74, 10.11 | 2.46×10–4 | 1.76×10–5 | 11 | 447 | 264 | 81,280 |
Cerebral palsy | 2.06 | 0.23, 7.53 | >0.99 | 0.433 | 1 | 161 | 274 | 81,566 |
OCD | 1.47 | 0.55, 3.11 | >0.99 | 0.406 | 5 | 966 | 270 | 80,761 |
Other neurodevelopmental disorder | 5.89 | 2.68, 11.86 | 6.41×10–4 | 4.58×10–5 | 11 | 406 | 264 | 81,321 |
Motor disorder | 2.00 | 0.93, 3.73 | >0.99 | 0.074 | 8 | 1,196 | 267 | 80,531 |
Anxiety | 1.14 | 0.89, 1.46 | >0.99 | 0.286 | 126 | 34,611 | 149 | 47,116 |
Depressive disorder | 1.47 | 1.15, 1.87 | 2.58×10–2 | 1.84×10–3 | 133 | 32,057 | 142 | 49,670 |
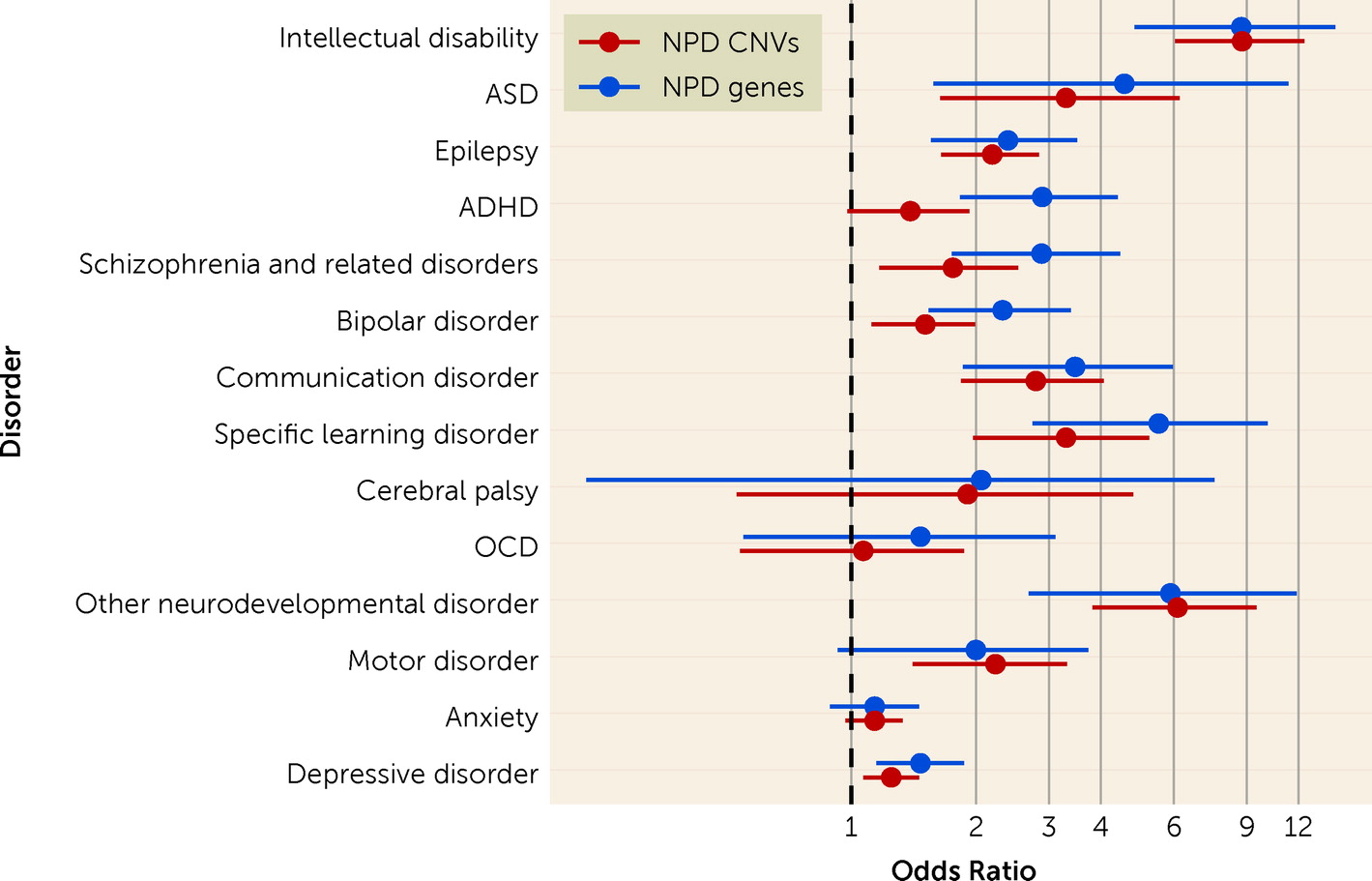
Sensitivity Analyses
Discussion
Conclusions
Acknowledgments
Supplementary Material
- View/Download
- 1.57 MB
References
Information & Authors
Information
Published In
History
Keywords
Authors
Author Contributions
Funding Information
Metrics & Citations
Metrics
Citations
Export Citations
If you have the appropriate software installed, you can download article citation data to the citation manager of your choice. Simply select your manager software from the list below and click Download.
For more information or tips please see 'Downloading to a citation manager' in the Help menu.
View Options
View options
PDF/EPUB
View PDF/EPUBLogin options
Already a subscriber? Access your subscription through your login credentials or your institution for full access to this article.
Personal login Institutional Login Open Athens loginNot a subscriber?
PsychiatryOnline subscription options offer access to the DSM-5-TR® library, books, journals, CME, and patient resources. This all-in-one virtual library provides psychiatrists and mental health professionals with key resources for diagnosis, treatment, research, and professional development.
Need more help? PsychiatryOnline Customer Service may be reached by emailing [email protected] or by calling 800-368-5777 (in the U.S.) or 703-907-7322 (outside the U.S.).