Using Claims Data to Generate Clinical Flags Predicting Short-Term Risk of Continued Psychiatric Hospitalizations
Abstract
Objective:
Methods:
Results:
Conclusions:
Methods
Medicaid claims data
Patterns of service use
Statistical analysis
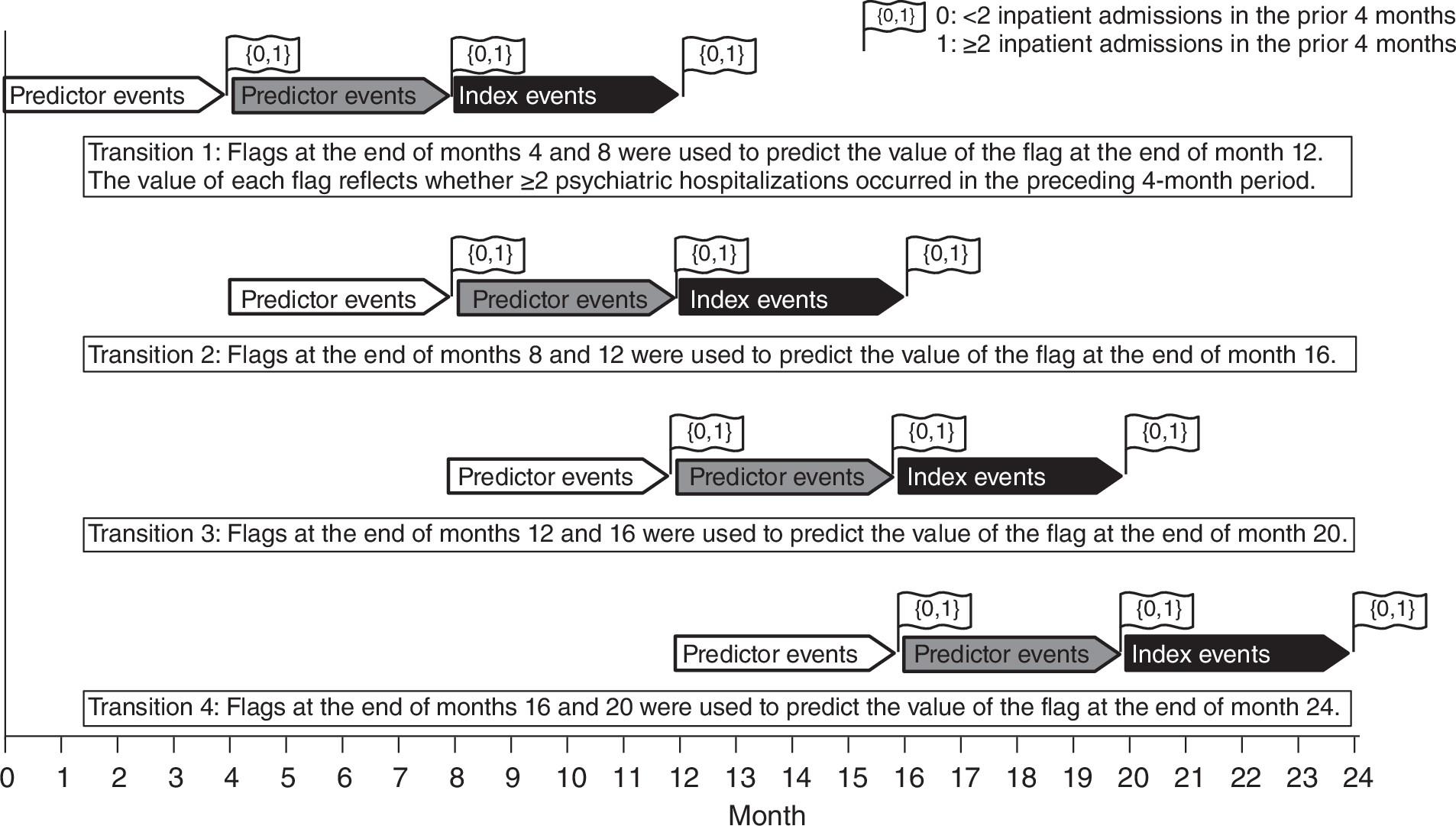
Results
Descriptive statistics
Observation month | Look-back period | Date of service count calculation | Had 90% Medicaid eligibility during look-back period | Used acute psychiatric inpatient care (%) | No outpatient visits (%) | No psychotropic drug fill (%)b | |||||
---|---|---|---|---|---|---|---|---|---|---|---|
Start | End | N.Y. | Penn. | N.Y. | Penn. | N.Y. | Penn. | N.Y. | Penn. | ||
1 | Dec. 1, 2008 | March 31, 2009 | April 1, 2009 | 10,205 | 1,680 | 28.3 | 6.9 | 23.6 | 20.2 | 36.4 | 24.6 |
2 | Jan. 1, 2009 | April 30, 2009 | May 1, 2009 | 10,145 | 1,619 | 24.9 | 6.3 | 23.8 | 21.9 | 36.3 | 25.3 |
3 | Feb. 1, 2009 | May 31, 2009 | June 1, 2009 | 10,000 | 1,567 | 20.8 | 5.4 | 25.2 | 23.6 | 37.4 | 27.2 |
4 | March 1, 2009 | June 30, 2009 | July 1, 2009 | 9,884 | 1,514 | 17.7 | 5.7 | 26.1 | 24.9 | 37.8 | 27.3 |
5 | April 1, 2009 | July 31, 2009 | Aug. 1, 2009 | 9,730 | 1,484 | 14.2 | 4.3 | 27.4 | 27.9 | 38.2 | 27.4 |
6 | May 1, 2009 | Aug. 31, 2009 | Sept. 1, 2009 | 9,604 | 1,449 | 13.4 | 3.4 | 28.2 | 28.8 | 38.2 | 29.3 |
7 | June 1, 2009 | Sept. 30, 2009 | Oct. 1, 2009 | 9,511 | 1,420 | 13.0 | 3.0 | 28.6 | 28.5 | 39.0 | 28.7 |
8 | July 1, 2009 | Oct. 31, 2009 | Nov. 1, 2009 | 9,405 | 1,386 | 12.5 | 3.2 | 29.1 | 30.5 | 38.0 | 28.4 |
9 | Aug. 1, 2009 | Nov. 30, 2009 | Dec. 1, 2009 | 9,313 | 1,375 | 11.9 | 3.2 | 29.4 | 30.8 | 37.7 | 29.5 |
10 | Sept. 1, 2009 | Dec. 31, 2009 | Jan. 1, 2010 | 9,206 | 1,361 | 11.3 | 2.5 | 29.6 | 32.0 | 38.0 | 30.4 |
11 | Oct. 1, 2009 | Jan. 31, 2010 | Feb. 1, 2010 | 9,172 | 1,353 | 10.9 | 2.7 | 29.1 | 32.6 | 37.2 | 30.7 |
12 | Nov. 1, 2009 | Feb. 28, 2010 | March 1, 2010 | 9,111 | 1,330 | 10.0 | 2.3 | 30.2 | 33.4 | 37.4 | 32.2 |
13 | Dec. 1, 2009 | March 31, 2010 | April 1, 2010 | 9,048 | 1,323 | 10.3 | 2.9 | 29.3 | 33.1 | 36.8 | 31.1 |
14 | Jan. 1, 2010 | April 30, 2010 | May 1, 2010 | 9,019 | 1,302 | 10.3 | 2.8 | 29.0 | 32.9 | 35.4 | 31.3 |
15 | Feb. 1, 2010 | May 31, 2010 | June 1, 2010 | 8,985 | 1,295 | 10.1 | 2.8 | 29.3 | 32.3 | 36.4 | 34.4 |
16 | March 1, 2010 | June 30, 2010 | July 1, 2010 | 8,957 | 1,292 | 10.8 | 2.6 | 28.9 | 30.3 | 36.4 | 34.2 |
17 | April 1, 2010 | July 31, 2010 | Aug. 1, 2010 | 8,926 | 1,298 | 10.2 | 2.8 | 29.1 | 32.4 | 36.7 | 34.1 |
18 | May 1, 2010 | Aug. 31, 2010 | Sept. 1, 2010 | 8,856 | 1,298 | 10.1 | 2.8 | 28.8 | 33.6 | 36.1 | 35.7 |
19 | June 1, 2010 | Sept. 30, 2010 | Oct. 1, 2010 | 8,811 | 1,286 | 10.3 | 2.9 | 28.5 | 32.6 | 36.2 | 36.0 |
20 | July 1, 2010 | Oct. 31, 2010 | Nov. 1, 2010 | 8,779 | 1,281 | 10.0 | 3.0 | 28.8 | 33.3 | 35.7 | 37.4 |
21 | Aug. 1, 2010 | Nov. 30, 2010 | Dec. 1, 2010 | 8,744 | 1,274 | 9.5 | 2.1 | 29.8 | 34.0 | 36.2 | 37.2 |
22 | Sept. 1, 2010 | Dec. 31, 2010 | Jan. 1, 2011 | 8,703 | 1,267 | 9.2 | 2.1 | 29.4 | 34.6 | 36.1 | 37.8 |
23 | Oct. 1, 2010 | Jan. 31, 2011 | Feb. 1, 2011 | 8,658 | 1,252 | 9.0 | 2.8 | 29.6 | 34.6 | 35.0 | 39.5 |
24 | Nov. 1, 2010 | Feb. 28, 2011 | March 1, 2011 | 8,651 | 1,245 | 8.4 | 2.7 | 29.9 | 35.3 | 35.9 | 40.3 |
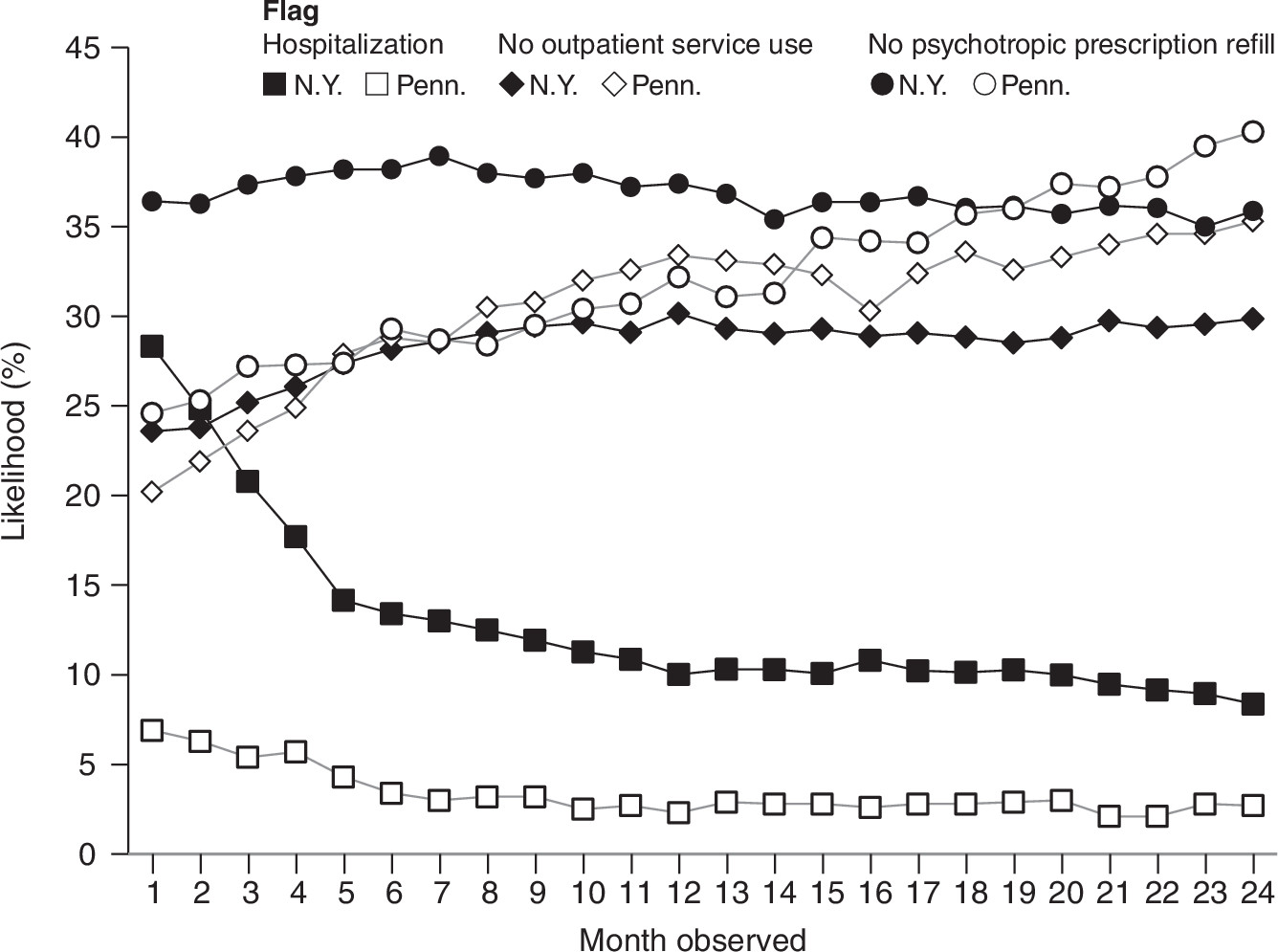
Predicting hospitalization from previous flags
Predicting hospitalization from other flags
Discussion
Conclusions
Acknowledgments and disclosures
References
Information & Authors
Information
Published In
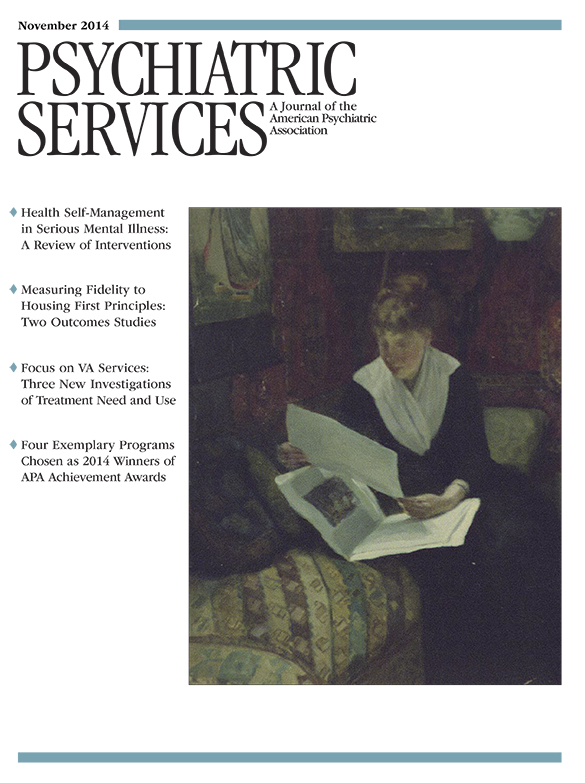
Cover: Woman Sitting on Couch Looking at Picture, by Alice Barber Stephens. Library of Congress, Prints and Photographs Division, DLC/PP-1933:0012.
History
Authors
Funding Information
Metrics & Citations
Metrics
Citations
Export Citations
If you have the appropriate software installed, you can download article citation data to the citation manager of your choice. Simply select your manager software from the list below and click Download.
For more information or tips please see 'Downloading to a citation manager' in the Help menu.
There are no citations for this item
View Options
View options
PDF/ePub
View PDF/ePubGet Access
Login options
Already a subscriber? Access your subscription through your login credentials or your institution for full access to this article.
Personal login Institutional Login Open Athens loginNot a subscriber?
PsychiatryOnline subscription options offer access to the DSM-5-TR® library, books, journals, CME, and patient resources. This all-in-one virtual library provides psychiatrists and mental health professionals with key resources for diagnosis, treatment, research, and professional development.
Need more help? PsychiatryOnline Customer Service may be reached by emailing [email protected] or by calling 800-368-5777 (in the U.S.) or 703-907-7322 (outside the U.S.).