Assessing the Long-Term Effectiveness of a Behavioral Health Home for Adults With Bipolar and Psychotic Disorders
Abstract
Objective:
Methods:
Results:
Conclusions:
Highlights
Methods
Setting and Intervention
Study Period and Sample
Statistical Analysis
Primary and Secondary Outcomes
Exploratory Subgroup Analyses
Results
Study Sample
Control group | Behavioral health home | |||||
---|---|---|---|---|---|---|
(N=1,929) | (N=413) | |||||
Characteristic | N | % | N | % | p | |
Age (M±SD) | 50.03±.39 | 50.74±.70 | .42 | |||
Female | 1,036 | 53.71 | 186 | 45.04 | <.01 | |
Native English speaker | 1,547 | 80.20 | 374 | 90.56 | <.01 | |
Diabetes | 344 | 17.83 | 78 | 18.89 | .62 | |
Primary psychotic disorder | 979 | 50.75 | 355 | 85.96 | <.01 | |
Bipolar disorder | 1,182 | 61.28 | 128 | 30.99 | <.01 | |
Race-ethnicity | ||||||
Asian | 70 | 3.63 | 18 | 4.36 | .48 | |
Hispanic | 168 | 8.71 | 6 | 1.94 | <.01 | |
Non-Hispanic Black | 302 | 15.66 | 95 | 23.00 | <.01 | |
Non-Hispanic White | 1,183 | 61.33 | 262 | 63.44 | .42 | |
Portuguese or Brazilian | 158 | 8.19 | 20 | 4.84 | .02 | |
Health insurance | ||||||
Medicaid | 1,027 | 53.24 | 181 | 43.83 | <.01 | |
Medicare | 737 | 38.21 | 209 | 50.61 | <.01 | |
Private | 156 | 8.09 | 23 | 5.57 | .08 | |
Self-pay | 8 | .41 | 0 | .19 | ||
Marital status | ||||||
Divorced | 216 | 11.20 | 33 | 7.99 | .05 | |
Legally separated | 67 | 3.47 | 5 | 1.21 | .02 | |
Married | 289 | 14.98 | 37 | 8.96 | <.01 | |
Single | 1,263 | 65.47 | 329 | 79.66 | <.01 | |
Widowed | 78 | 4.04 | 9 | 2.18 | .07 | |
Area-level indicators (M±SD %) | ||||||
Female-headed households | 14.62±.19 | 14.85±.37 | .60 | |||
Less than a high school education | 13.75±.22 | 10.21±.38 | <.01 | |||
Foreign born | 30.66±.26 | 27.97±.51 | <.01 | |||
Living in poverty | 13.87±.15 | 13.43±.30 | .23 |
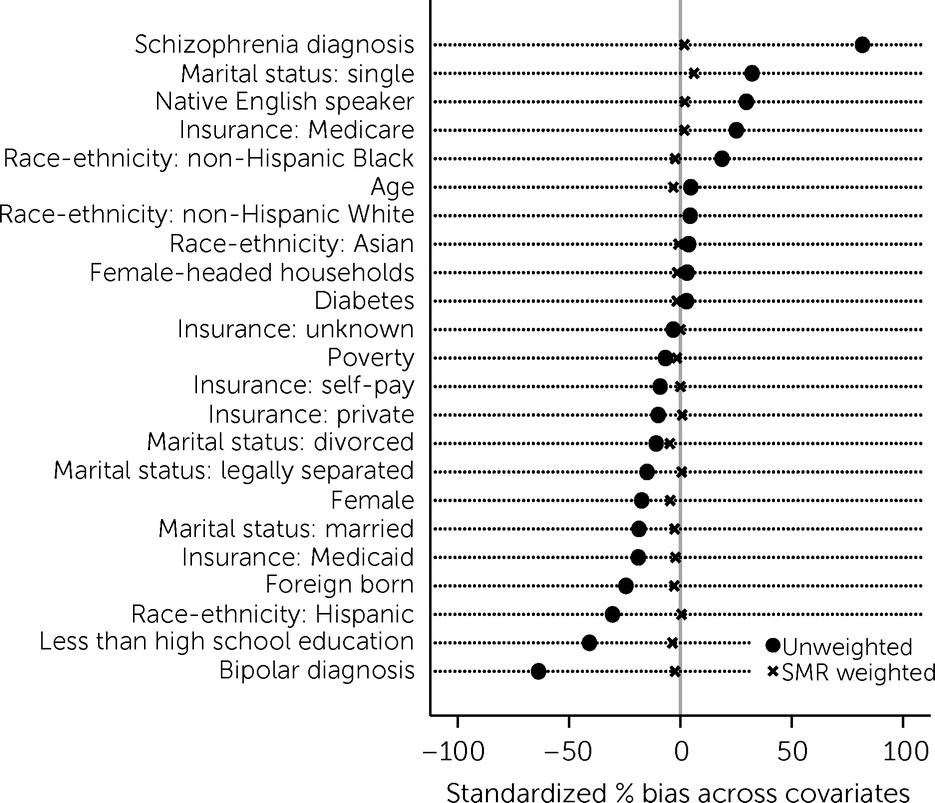
Service Utilization DID
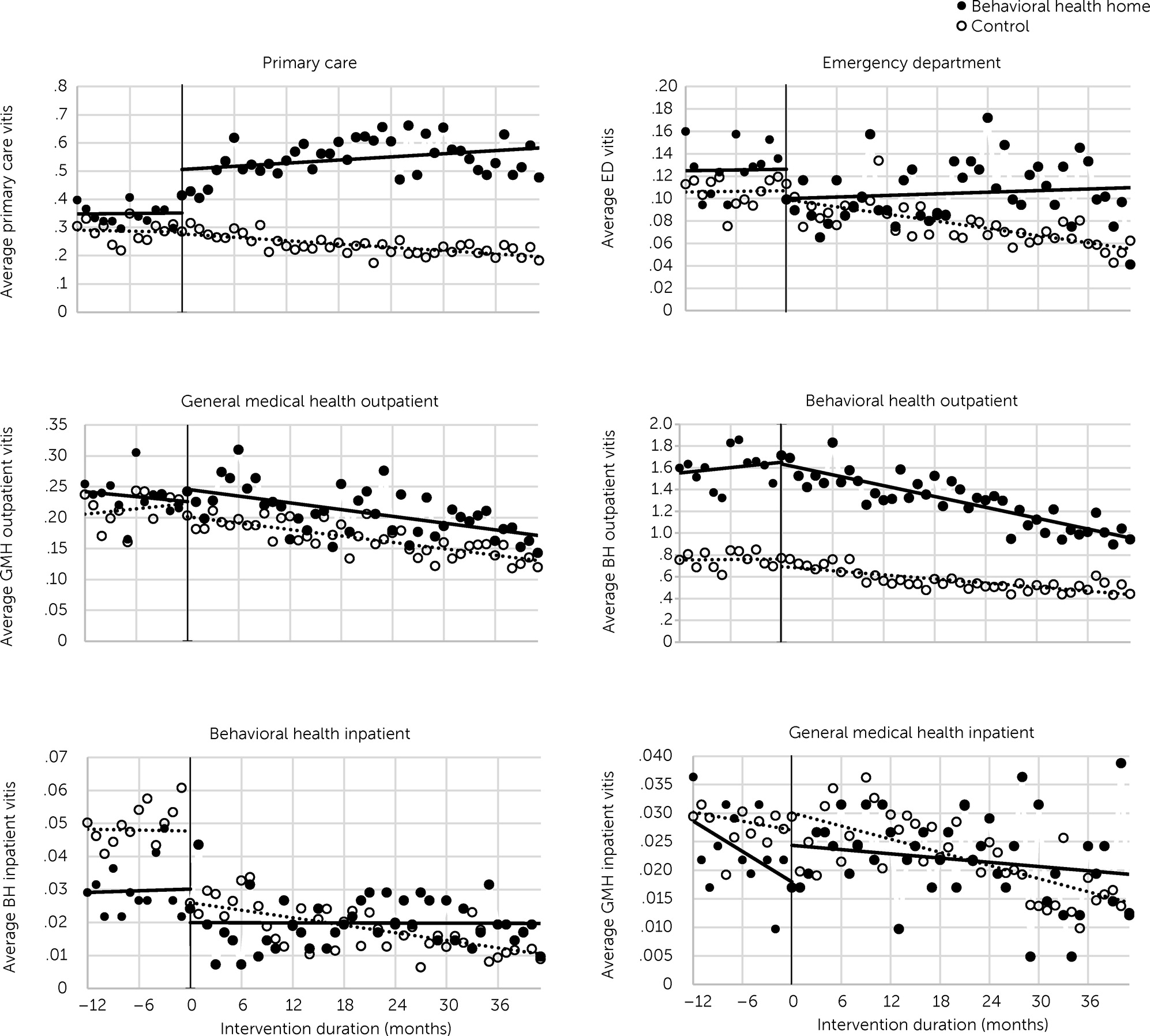
Pre-post intervention group shifts | Difference-in-difference shifts | |||||||||||
---|---|---|---|---|---|---|---|---|---|---|---|---|
(for contextualization) | (primary outcomes)b | |||||||||||
BHH level shift | BHH trend shift | Control level shift | Control trend shift | Level shift | Trend shift | |||||||
Visit type | Value | SE | Value | SE | Value | SE | Value | SE | Value | SE | Value | SE |
Primary care | .20** | −.03 | .008 | −.005 | .004 | −.03 | .0003 | −.004 | .18** | −.04 | .006 | −.004 |
ED | −.03* | −.01 | −.00009 | −.002 | .003 | −.008 | .0004 | −.001 | −.03* | −.01 | −.0002 | −.002 |
GMH inpatient | .006 | −.005 | .0007 | −.0006 | .004 | −.003 | −.00003 | −.0003 | .002 | −.003 | .0007** | −.0002 |
GMH outpatient | .06** | −.01 | .005** | −.001 | −.01 | −.01 | −.002 | −.001 | .06** | −.01 | .004** | −.001 |
Behavioral health inpatient | −.01 | −.008 | −.0003 | −.0009 | −.03** | −.004 | −.001** | −.0005 | .02* | −.008 | .001 | −.0008 |
Behavioral health outpatient | −.03 | −.05 | −.03** | −.005 | −.09* | −.04 | −.009** | −.003 | .07 | −.05 | −.02** | −.004 |
Subgroup Analyses
Cardiometabolic Measures
Discussion
Study Strengths
Study Limitations
Conclusions
Footnote
Supplementary Material
- View/Download
- 595.80 KB
References
Information & Authors
Information
Published In
History
Keywords
Authors
Competing Interests
Funding Information
Metrics & Citations
Metrics
Citations
Export Citations
If you have the appropriate software installed, you can download article citation data to the citation manager of your choice. Simply select your manager software from the list below and click Download.
For more information or tips please see 'Downloading to a citation manager' in the Help menu.
View Options
View options
PDF/EPUB
View PDF/EPUBLogin options
Already a subscriber? Access your subscription through your login credentials or your institution for full access to this article.
Personal login Institutional Login Open Athens loginNot a subscriber?
PsychiatryOnline subscription options offer access to the DSM-5-TR® library, books, journals, CME, and patient resources. This all-in-one virtual library provides psychiatrists and mental health professionals with key resources for diagnosis, treatment, research, and professional development.
Need more help? PsychiatryOnline Customer Service may be reached by emailing [email protected] or by calling 800-368-5777 (in the U.S.) or 703-907-7322 (outside the U.S.).