Investigating the Contribution of Common Genetic Variants to the Risk and Pathogenesis of ADHD
Abstract
Objective:
Method:
Results:
Conclusions:
Method
Subjects and Clinical Measures
Diagnosis | N | % |
---|---|---|
ADHD diagnoses (lifetime)a | ||
DSM-IV ADHD, combined type | 498 | 64.8 |
DSM-IV ADHD, predominantly inattentive type | 162 | 21.1 |
DSM-IV ADHD, predominantly hyperactive-impulsive type | 62 | 8.1 |
DSM-III-R ADHD | 46 | 6.0 |
Other diagnoses (current) | ||
DSM-IV conduct disorder | 107 | 13.7 |
DSM-IV oppositional defiant disorder | 364 | 46.5 |
DSM-IV anxiety disorder (generalized anxiety disorder, separation anxiety, or social phobia) | 37 | 4.7 |
DSM-IV depressive disorder (any) | 22 | 2.8 |
Genotyping
Quality Control Assessment
CNV Data
Statistical Analysis
GWAS.
Pathway analysis of Cardiff GWAS data.
Overlap of GWAS and CNV pathways.
Results
GWAS
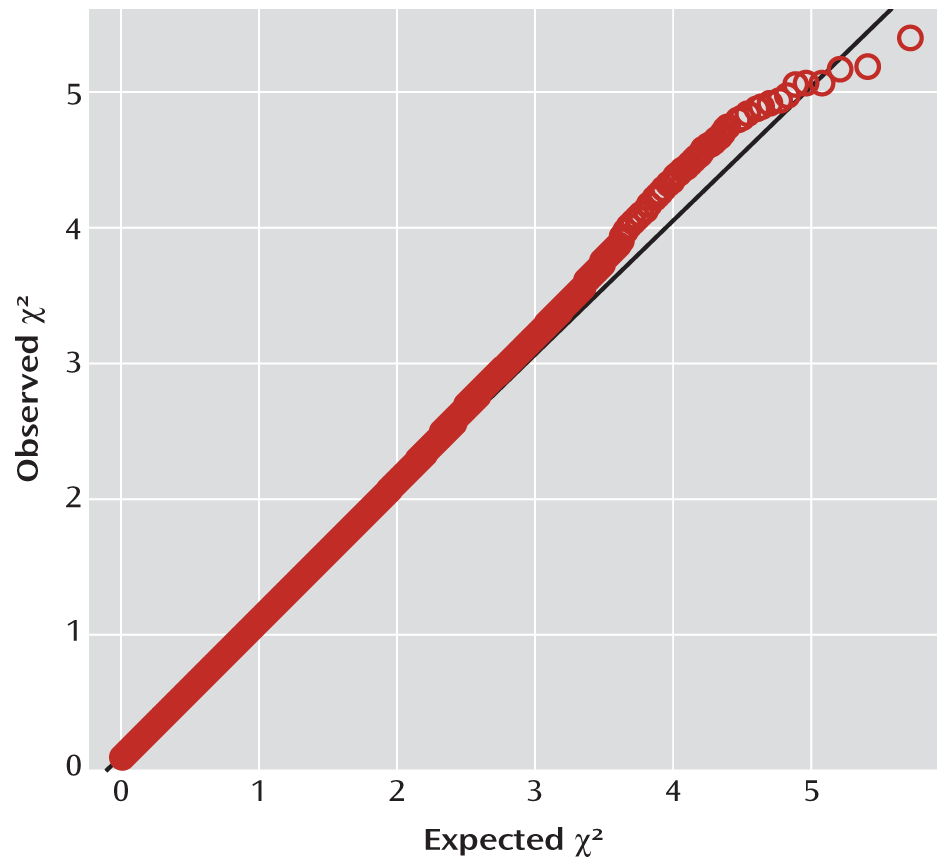
Minor Allele | ||||||||||
---|---|---|---|---|---|---|---|---|---|---|
SNP | Chromosome | Position | Closest Gene | Location Relative to Gene | Minor Allele | Other Allele | Minor Allele Frequency | p | Odds Ratio | 95% CI |
rs1744062 | 6 | 137350879 | IL20RA | Within noncoding gene | G | A | 0.43 | 4.16E-06 | 0.75 | 0.67–0.85 |
rs11079828 | 17 | 43964102 | HOXB1 | Upstream | T | C | 0.47 | 6.54E-06 | 1.32 | 1.17–1.49 |
rs42259 | 5 | 14439655 | TRIO | Intronic | T | C | 0.17 | 6.76E-06 | 1.41 | 1.22–1.64 |
rs3779312 | 7 | 77549692 | MAGI2 | Intronic | T | C | 0.21 | 8.38E-06 | 1.37 | 1.19–1.57 |
rs616668 | 12 | 110458663 | ATXN2 | Intronic | G | T | 0.20 | 8.62E-06 | 1.38 | 1.20–1.59 |
rs11175219 | 12 | 62648986 | SRGAP1 | Intronic | T | C | 0.12 | 1.06E-05 | 1.46 | 1.23–1.73 |
rs4238186 | 13 | 18588836 | LOC100128765 | Intergenic | A | G | 0.18 | 1.11E-05 | 1.39 | 1.20–1.61 |
rs7746680 | 6 | 45885325 | - | Intergenic | A | G | 0.24 | 1.14E-05 | 1.35 | 1.18–1.54 |
rs11686538 | 2 | 225526808 | DOCK10 | Intronic | G | A | 0.29 | 1.27E-05 | 0.74 | 0.64–0.84 |
rs1304358 | 2 | 198677828 | PLCL1 | Intronic | C | T | 0.49 | 1.27E-05 | 1.30 | 1.16–1.47 |
rs406742 | 10 | 8885947 | - | Intergenic | G | A | 0.26 | 1.49E-05 | 1.33 | 1.17–1.51 |
rs790531 | 13 | 49623515 | DLEU2 | Within noncoding gene | G | A | 0.06 | 1.50E-05 | 1.62 | 1.30–2.02 |
rs6815704 | 4 | 93693589 | GRID2 | Intronic | A | G | 0.15 | 2.09E-05 | 1.40 | 1.20–1.64 |
rs9842394 | 3 | 181095930 | PEX5L | Intronic | T | C | 0.47 | 2.68E-05 | 0.77 | 0.69–0.87 |
rs2636788 | 10 | 98866931 | SLIT1 | Intronic | G | A | 0.17 | 2.74E-05 | 0.70 | 0.59–0.83 |
rs1490046 | 5 | 173888653 | - | Intergenic | A | G | 0.08 | 2.87E-05 | 1.56 | 1.27–1.92 |
rs1050567 | 2 | 61559167 | XPO1 | 3′ untranslated region | T | C | 0.11 | 2.89E-05 | 1.44 | 1.22–1.72 |
rs9384245 | 6 | 155201820 | TIAM2 | Intronic | T | C | 0.42 | 3.00E-05 | 0.77 | 0.68–0.87 |
rs1370072 | 13 | 54739939 | - | Intergenic | T | C | 0.45 | 3.28E-05 | 1.29 | 1.14–1.45 |
rs874836 | 22 | 15681843 | XKR3 | Intronic | A | G | 0.13 | 3.32E-05 | 1.41 | 1.20–1.67 |
Pathway Analysis of Cardiff SNP Data
Overlap of Enriched Pathways Between CNV and SNP Data
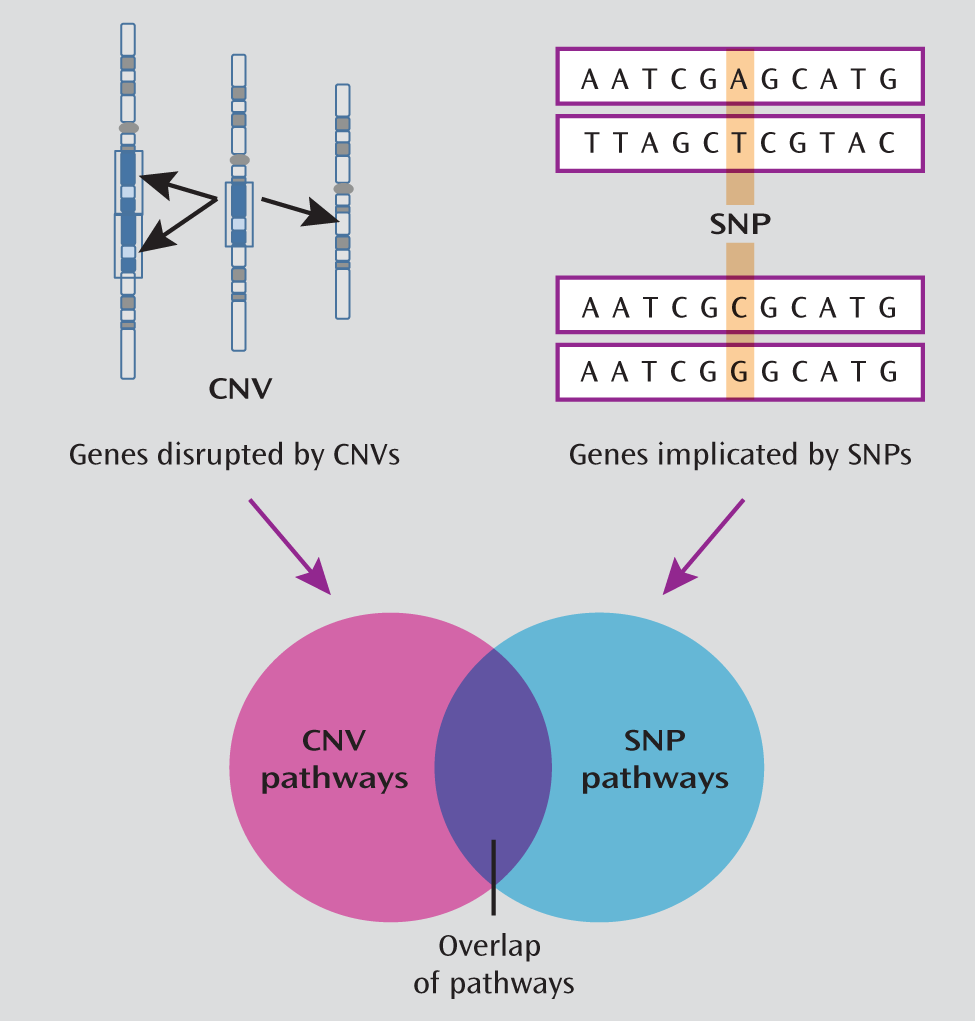
Pathway Numbera | Number of Genes | Gene Hits (Cases) | Gene Hits (Comparison) | p (CNV) | p (corr)b | p (GWAS) | Description |
---|---|---|---|---|---|---|---|
MGI:5278 | 188 | 14 | 0 | 1.47E-05 | 0.002 | 0.030 | Abnormal cholesterol homeostasis |
MGI:3947 | 182 | 13 | 0 | 2.61E-05 | 0.004 | 0.023 | Abnormal cholesterol level |
MGI:180 | 169 | 13 | 0 | 2.61E-05 | 0.004 | 0.026 | Abnormal circulating cholesterol level |
GO:16746 | 214 | 14 | 0 | 1.42E-04 | 0.009 | 0.004 | Transferase activity, transferring acyl groups |
GO:8415 | 203 | 13 | 0 | 1.43E-04 | 0.009 | 0.003 | Acyltransferase activity |
GO:16747 | 205 | 13 | 0 | 1.43E-04 | 0.008 | 0.004 | Transferase activity, transferring acyl groups other than amino-acyl groups |
GO:51298 | 11 | 8 | 0 | 6.95E-04 | 0.050 | 0.033 | Centrosome duplication |
GO:32680 | 34 | 7 | 1 | 8.83E-03 | 0.341 | 0.014 | Regulation of tumor necrosis factor production |
GO:5261 | 271 | 17 | 6 | 1.84E-02 | 0.547 | 0.042 | Cation channel activity |
GO:7417 | 441 | 28 | 10 | 2.77E-02 | 0.683 | 0.002 | Central nervous system development |
GO:16247 | 56 | 8 | 2 | 3.07E-02 | 0.719 | 0.026 | Channel regulator activity |
GO:8233 | 572 | 25 | 8 | 4.89E-02 | 0.848 | 0.037 | Peptidase activity |
GO:70011 | 553 | 25 | 8 | 4.89E-02 | 0.848 | 0.038 | Peptidase activity, acting on L-amino acid peptides |
Discussion
Acknowledgments
Footnotes
Supplementary Material
- View/Download
- 488.80 KB
References
Information & Authors
Information
Published In
History
Authors
Author Contributions
Funding Information
Metrics & Citations
Metrics
Citations
Export Citations
If you have the appropriate software installed, you can download article citation data to the citation manager of your choice. Simply select your manager software from the list below and click Download.
For more information or tips please see 'Downloading to a citation manager' in the Help menu.
View Options
View options
PDF/EPUB
View PDF/EPUBGet Access
Login options
Already a subscriber? Access your subscription through your login credentials or your institution for full access to this article.
Personal login Institutional Login Open Athens loginNot a subscriber?
PsychiatryOnline subscription options offer access to the DSM-5-TR® library, books, journals, CME, and patient resources. This all-in-one virtual library provides psychiatrists and mental health professionals with key resources for diagnosis, treatment, research, and professional development.
Need more help? PsychiatryOnline Customer Service may be reached by emailing [email protected] or by calling 800-368-5777 (in the U.S.) or 703-907-7322 (outside the U.S.).