Auditory Oddball Responses Across the Schizophrenia-Bipolar Spectrum and Their Relationship to Cognitive and Clinical Features
Abstract
Objective:
Methods:
Results:
Conclusions:
METHODS
Recruitment
Group | Analysis | |||||||||||||
---|---|---|---|---|---|---|---|---|---|---|---|---|---|---|
Characteristic | Healthy Comparison (N=415) | Bipolar I Disorder Without Psychotic Features (N=66) | Bipolar I Disorder With Psychotic Features (N=171) | Schizoaffective Disorder (N=201) | Schizophrenia (N=225) | Test | df | p | Post Hoc Tukey’s B | |||||
N | % | N | % | N | % | N | % | N | % | χ2 | df | p | ||
Sex | 31.7 | 4 | <0.001 | |||||||||||
Male | 178 | 43 | 19 | 29 | 78 | 46 | 91 | 45 | 139 | 62 | ||||
Female | 137 | 57 | 47 | 71 | 93 | 54 | 110 | 55 | 86 | 38 | ||||
Mean | SD | Mean | SD | Mean | SD | Mean | SD | Mean | SD | F | df | p | ||
Age (years) | 34.74 | 12.5 | 40.39 | 12.16 | 37.2 | 11.72 | 40.32 | 10.98 | 40.03 | 11.66 | 11.89 | 4, 1073 | <0.001 | |
N | % | N | % | N | % | N | % | N | % | χ2 | df | p | ||
Site | 99.27 | 16 | <0.001 | |||||||||||
Boston | 40 | 29.9 | 25 | 33.8 | 15 | 25 | 15 | 25 | 15 | 25 | ||||
Chicago | 55 | 41 | 29 | 39.2 | 26 | 43.3 | 26 | 43.3 | 26 | 43.3 | ||||
Dallas | 7 | 5.2 | 4 | 5.4 | 3 | 5 | 3 | 5 | 3 | 5 | ||||
Georgia | 21 | 15.7 | 9 | 12.2 | 12 | 20 | 12 | 20 | 12 | 20 | ||||
Hartford | 11 | 8.2 | 7 | 9.5 | 4 | 6.7 | 4 | 6.7 | 4 | 6.7 | ||||
Ethnicity | 4.64 | 4 | 0.33 | |||||||||||
Hispanic | 53 | 13 | 9 | 14 | 32 | 19 | 32 | 16 | 27 | 12 | ||||
Non-Hispanic | 353 | 87 | 57 | 86 | 138 | 81 | 167 | 84 | 194 | 88 | ||||
Race | 112.87 | 28 | <0.001 | |||||||||||
Black or African American | 113 | 28 | 14 | 21 | 44 | 26 | 77 | 39 | 118 | 53 | ||||
American Indian | 1 | 0 | 0 | 0 | 1 | 1 | 1 | 1 | 2 | 1 | ||||
Asian | 33 | 8 | 0 | 0 | 1 | 1 | 8 | 4 | 10 | 5 | ||||
White or Caucasian | 227 | 56 | 45 | 68 | 108 | 64 | 84 | 42 | 66 | 30 | ||||
Native Hawaiian | 1 | 0 | 1 | 2 | 0 | 0 | 0 | 0 | 0 | 0 | ||||
Multiracial | 18 | 4 | 4 | 6 | 10 | 6 | 22 | 11 | 16 | 7 | ||||
Other/ unknown | 14 | 3 | 2 | 3 | 6 | 4 | 8 | 4 | 10 | 5 | ||||
Mean | SD | Mean | SD | Mean | SD | Mean | SD | Mean | SD | F | df | p | ||
Illness duration (years) | NA | NA | 23.98 | 12.48 | 19.04 | 12.51 | 23.16 | 12.48 | 21.02 | 11.87 | 4.31 | 3, 621 | 0.01 | BDP/SZ < SZ/SAD/BDNP |
Years of formal educationb | 15.29 | 2.29 | 14.31 | 3.12 | 14.5 | 2.41 | 13.31 | 2.26 | 12.84 | 2.33 | 46.59 | 4, 1033 | <0.001 | SZ/SAD < BDNP/BDP < HC |
17.46 | 3, 635 | <0.001 | ||||||||||||
Number of trials in each waveform average | ||||||||||||||
Standard | 542 | 41.77 | 545 | 32.68 | 540 | 42.88 | 533 | 48.39 | 534 | 46.77 | 2.43 | 4, 1073 | 0.05 | n.s. |
Target | 96 | 6.91 | 94 | 8.92 | 95 | 7.95 | 94 | 8.92 | 94 | 8.89 | 2.66 | 4, 1074 | 0.03 | n.s. |
GAF scaleb | 83.42 | 6.61 | 57.88 | 11.4 | 56.73 | 13.47 | 53.26 | 11.15 | 50.51 | 11.54 | 456.38 | 4, 942 | <0.001 | SZ/SAD < BDP/BDNP < HC |
10.84 | 3, 606 | <0.001 | ||||||||||||
Birchwood Social Functioning Scaleb | 154.15 | 17.34 | 130.15 | 20.41 | 131.11 | 23.65 | 116.78 | 22.93 | 117.33 | 21.32 | 158.54 | 4, 985 | <0.001 | SAD/SZ < BDNP/BDP < HC |
17.82 | 3, 605 | <0.001 | ||||||||||||
BACSb verbal memory score | –0.29 | 1.15 | –1.21 | 1.31 | –0.77 | 1.32 | –1.36 | 1.33 | –1.5 | 1.32 | 41.94 | 4, 1008 | <0.001 | SZ/SAD/BDNP < BDP < HC |
10.64 | 3, 643 | <0.001 | ||||||||||||
BACS digit sequencing score | –0.33 | 1.01 | –0.64 | 1.13 | –0.68 | 1.17 | –0.97 | 1.04 | –1.33 | 1.18 | 31.93 | 4, 1007 | <0.001 | SZ < SAD/BDP < BDP/BDNP < HC |
12.98 | 3, 642 | <0.001 | ||||||||||||
BACS token motor score | –0.62 | 1.15 | –0.97 | 1.17 | –1.35 | 1.03 | –1.41 | 1.09 | –1.62 | 1.17 | 33.68 | 4, 986 | <0.001 | SZ/SAD/BDP < BDNP < HC |
5.77 | 3, 623 | 0.001 | ||||||||||||
BACS verbal fluency score | 0.13 | 1.16 | –0.31 | 1.25 | –0.3 | 1.07 | –0.58 | 1.08 | –0.71 | 1.17 | 23.49 | 4, 1006 | <0.001 | SZ/SAD < SAD/BDNP/BDP < HC |
5.08 | 3, 642 | 0.002 | ||||||||||||
BACS symbol coding score | –0.11 | 1.07 | –0.75 | 1.01 | –0.77 | 1.08 | –1.13 | 1.17 | –1.4 | 1.07 | 57.02 | 4, 1007 | <0.001 | SZ/SAD < BDP/BDNP < HC |
12.62 | 3, 642 | <0.001 | ||||||||||||
BACS Tower of London score | –0.1 | 1.13 | –0.48 | 1.16 | –0.32 | 1.12 | –0.65 | 1.31 | –0.97 | 1.38 | 18.96 | 4, 1008 | <0.001 | SZ/SAD < SAD/BDNP/BDP < BDP/HC |
8.75 | 3, 643 | <0.001 | ||||||||||||
PANSS positive score | NA | NA | 12.76 | 3.64 | 13.82 | 5.86 | 17.85 | 6.92 | 17.71 | 6.29 | 22.62 | 3, 592 | <0.001 | BDNP/BDP < SZ/SAD |
PANSS negative score | NA | NA | 14.57 | 5.86 | 13.85 | 6.44 | 16.32 | 7.16 | 17.39 | 6.71 | 9.22 | 3, 591 | <0.001 | BDP/BDNP < BDNP/SAD < SAD/SZ |
PANSS general score | NA | NA | 31.67 | 8.83 | 31.06 | 10.59 | 33.16 | 9.87 | 32.24 | 10 | 1.28 | 3, 592 | 0.28 | n.s. |
PANSS total score | NA | NA | 59 | 15.84 | 58.72 | 21.12 | 67.33 | 21.39 | 67.42 | 20.15 | 8.18 | 3, 591 | <0.001 | BDP/BDNP < SAD/SZ |
MADRS score | NA | NA | 14.76 | 9.33 | 14.56 | 11.82 | 14.01 | 10.7 | 9.75 | 8.94 | 8.90 | 3, 600 | <0.001 | SZ < SAD/BDP/BDNP |
YMRS score | NA | NA | 9.41 | 7.1 | 8.98 | 8.82 | 10.83 | 7.95 | 9.93 | 7.28 | 1.64 | 3, 599 | 0.18 | n.s. |
Stimuli
EEG Recording and Preprocessing


ERP Principal Component Analysis for Spatial Data Reduction
Event-Related Oscillation Time-Frequency Analysis
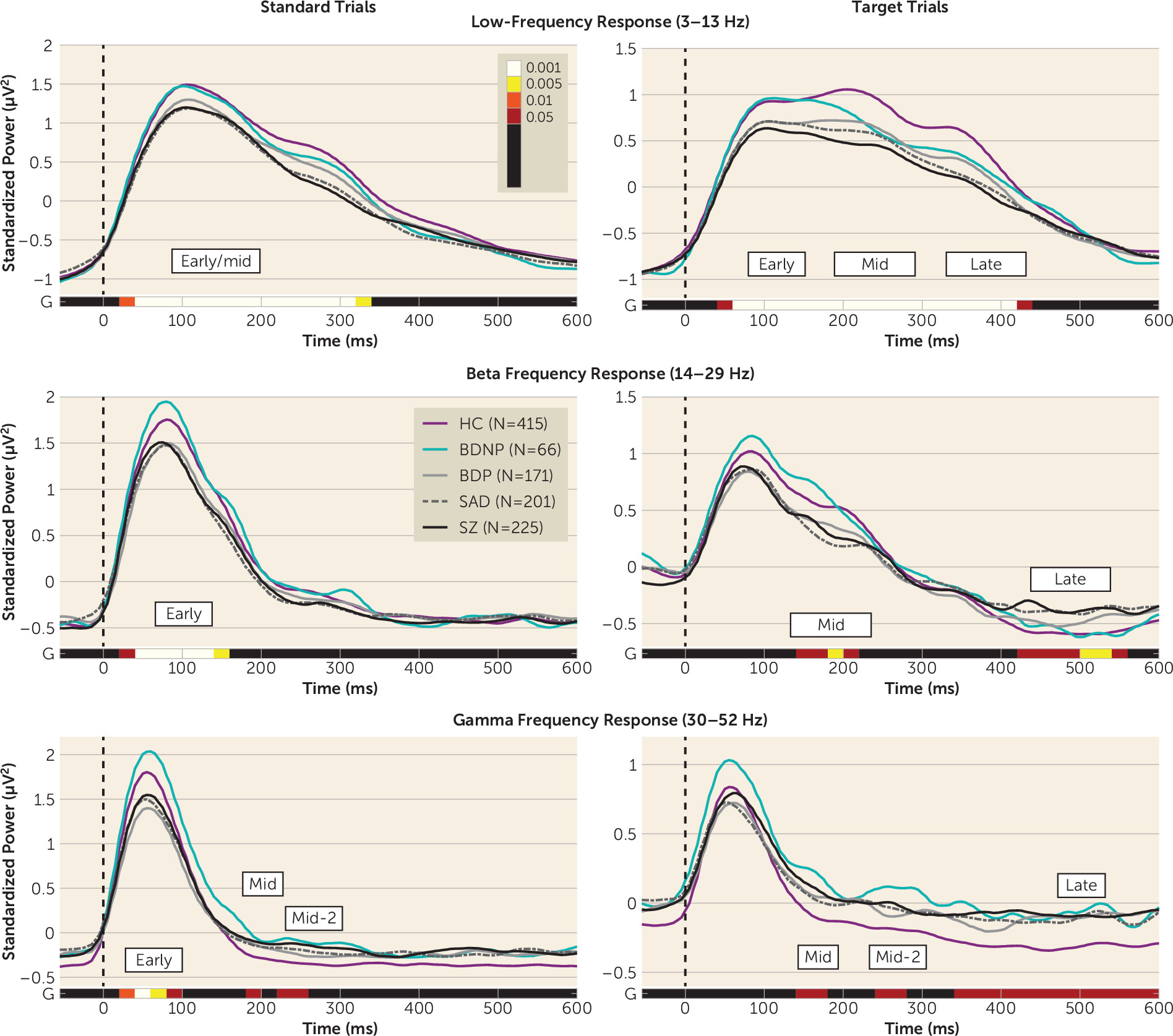
Age Adjustment
Statistical Analysis
Comparison of B-SNIP1 and B-SNIP2.
B-SNIP2 sample only analyses.
Group Discrimination Analyses
Canonical Correlation Analyses for Clinical Features and Cognition
Medication Association Analysis
Results
B-SNIP1 Compared With B-SNIP2 Voltage Virtual Sensors for Standard and Target Trials
Voltage Virtual Sensors for Standard and Target Trials
Frequency Bands for Standard and Target Trials
Multivariate Analyses
Canonical discriminant analysis.

Canonical Correlation Analysis Relating Neural Responses and Cognitive Performance
Canonical Correlation Analysis Relating Neural Responses and Clinical Features
Discussion
Acknowledgments
Supplementary Material
- Download
- 830.74 KB
References
Information & Authors
Information
Published In
History
Keywords
Authors
Competing Interests
Funding Information
Metrics & Citations
Metrics
Citations
Export Citations
If you have the appropriate software installed, you can download article citation data to the citation manager of your choice. Simply select your manager software from the list below and click Download.
For more information or tips please see 'Downloading to a citation manager' in the Help menu.
There are no citations for this item
View Options
View options
PDF/ePub
View PDF/ePubGet Access
Login options
Already a subscriber? Access your subscription through your login credentials or your institution for full access to this article.
Personal login Institutional Login Open Athens loginNot a subscriber?
PsychiatryOnline subscription options offer access to the DSM-5-TR® library, books, journals, CME, and patient resources. This all-in-one virtual library provides psychiatrists and mental health professionals with key resources for diagnosis, treatment, research, and professional development.
Need more help? PsychiatryOnline Customer Service may be reached by emailing [email protected] or by calling 800-368-5777 (in the U.S.) or 703-907-7322 (outside the U.S.).