Neural Predictors of Initiating Alcohol Use During Adolescence
Abstract
Objective:
Method:
Results:
Conclusions:
Method
Participants
Variable | Continuous Nonusers (N=67) | Moderate to Heavy Alcohol Initiators (N=70) | ||||||||
---|---|---|---|---|---|---|---|---|---|---|
Mean | SD | % | Mean | SD | % | t or χ2 | df | p | Cohen’s d | |
Demographic and family variables | ||||||||||
Sex (female) | 60 | 29 | 13.48 | 1, N=137 | <0.001 | 0.661 | ||||
Follow-up age (range=16–19)b | 18.20 | 0.66 | 18.20 | 0.57 | 0.07 | 135 | 0.943 | 0.000 | ||
Race (Caucasian) | 58 | 77 | 5.63 | 1, N=137 | 0.018 | 0.414 | ||||
Baseline Hollingshead Index of Social Position score (socioeconomic status) | 24.03 | 16.75 | 21.11 | 11.50 | 1.19 | 135 | 0.235 | 0.203 | ||
Family history density of alcohol or drug use disorder | 0.17 | 0.28 | 0.18 | 0.31 | 0.14 | 135 | 0.887 | 0.034 | ||
Baseline Pubertal Development Scale score (girls only; N=60) | 15.05 | 3.15 | 14.95 | 3.15 | 0.12 | 58 | 0.908 | 0.032 | ||
Baseline Pubertal Development Scale score (boys only; N=77) | 10.81 | 3.33 | 11.20 | 3.37 | 0.48 | 75 | 0.632 | 0.116 | ||
Baseline grade in school | 6.76 | 0.74 | 7.00 | 0.87 | 1.73 | 135 | 0.086 | 0.297 | ||
Birth order | 1.52 | 0.79 | 1.64 | 0.76 | 0.91 | 135 | 0.364 | 0.155 | ||
Baseline % living with both parents | 79 | 81 | 0.12 | 1, N=137 | 0.732 | 0.059 | ||||
Baseline % youth with biological parents married to each other | 78 | 77 | 0.00 | 1, N=137 | 0.948 | 0.000 | ||||
Baseline youth behavior, mood, and cognition | ||||||||||
Initiated dating by age 14 (%) | 22 | 61 | 21.37 | 1, N=137 | <0.001 | 0.860 | ||||
Involved in extracurricular activities (%) | 82 | 89 | 1.15 | 1, N=137 | 0.283 | 0.184 | ||||
Hours of video games played per week | 3.10 | 4.95c | 2.47 | 4.04c | 0.72 | 106 | 0.472 | 0.139 | ||
Grade point average (4.0 scale) | 3.65 | 0.45 | 3.47 | 0.55 | 2.14 | 135 | 0.034 | 0.358 | ||
CBCL externalizing disorder t score | 41.09 | 7.12d | 40.42 | 7.64d | 0.52 | 128 | 0.606 | 0.091 | ||
CBCL internalizing t score | 44.48 | 8.80d | 42.11 | 7.65d | 1.65 | 128 | 0.102 | 0.287 | ||
CBCL withdrawn t score | 52.00 | 3.79d | 51.42 | 2.69d | 1.00 | 128 | 0.319 | 0.176 | ||
CBCL somatic complaints t score | 52.45 | 4.13d | 51.79 | 3.15d | 1.04 | 128 | 0.302 | 0.180 | ||
CBCL anxious/depressed t score | 51.61 | 3.60d | 51.11 | 2.60d | 0.92 | 128 | 0.361 | 0.159 | ||
CBCL social problems t score | 51.33 | 3.62d | 50.55 | 1.64d | 1.60 | 128 | 0.113 | 0.278 | ||
CBCL thought problems t score | 51.98 | 3.90d | 51.03 | 2.31d | 1.70 | 128 | 0.091 | 0.296 | ||
CBCL attention problems t score | 51.05 | 2.16d | 51.20 | 2.81d | 0.34 | 128 | 0.734 | 0.060 | ||
CBCL delinquent behavior t score | 50.59 | 1.84d | 50.98 | 2.53d | 1.01 | 128 | 0.317 | 0.176 | ||
CBCL aggressive behavior t score | 50.75 | 2.06d | 50.68 | 2.11d | 0.19 | 128 | 0.852 | 0.034 | ||
CBCL total problem t score | 39.83 | 9.75d | 37.83 | 8.83d | 1.22 | 128 | 0.223 | 0.215 | ||
Conduct Disorder Questionnaire total score | 0.45 | 1.08 | 1.17 | 1.88 | 2.75 | 135 | 0.007 | 0.470 | ||
Beck Depression Inventory–II total score | 1.39 | 2.75e | 1.25 | 2.81e | 0.30 | 132 | 0.765 | 0.050 | ||
State-Trait Anxiety Inventory total score | 26.22 | 6.66f | 26.68 | 6.48f | 0.40 | 130 | 0.690 | 0.070 | ||
AEQ total score | 79.14 | 32.23 | 92.45 | 29.75 | 2.51 | 135 | 0.013 | 0.429 | ||
AEQ global positive score | 37.81 | 8.37 | 40.83 | 9.53 | 1.97 | 135 | 0.051 | 0.336 | ||
AEQ social behavior change score | 36.92 | 7.41 | 41.23 | 7.94 | 3.28 | 135 | 0.001 | 0.561 | ||
AEQ improved performance score | 16.55 | 4.57 | 16.96 | 4.98 | 0.50 | 135 | 0.621 | 0.086 | ||
AEQ sexual enhancement score | 20.15 | 5.06 | 21.00 | 4.07 | 1.09 | 135 | 0.279 | 0.185 | ||
AEQ impaired performance score | 98.41 | 10.08 | 96.39 | 10.28 | 1.16 | 135 | 0.247 | 0.198 | ||
AEQ increased arousal score | 24.24 | 5.44 | 24.96 | 4.78 | 0.82 | 135 | 0.414 | 0.141 | ||
AEQ relaxation score | 41.88 | 9.83 | 43.87 | 7.90 | 1.31 | 135 | 0.193 | 0.293 | ||
Baseline substance use | ||||||||||
CDDR baseline lifetime smoking days | 0.03 | 0.17 | 0.11 | 0.65 | 1.03 | 135 | 0.305 | 0.168 | ||
CDDR baseline lifetime drinking days | 0.00 | 0.00 | 0.31 | 1.50 | 1.72 | 135 | 0.089 | 0.292 | ||
CDDR baseline lifetime marijuana use days | 0.00 | 0.00 | 0.09 | 0.44 | 1.59 | 135 | 0.115 | 0.289 | ||
Total number of repetitions excluded during functional magnetic resonance imaging | 4.46 | 6.77 | 7.03 | 8.82 | 1.90 | 134 | 0.060 | 0.327 | ||
Follow-up substance use | ||||||||||
Age at first use of alcohol | 16.54 | 1.27g | 15.46 | 1.60 | 3.09 | 94 | 0.003 | 0.748 | ||
Lifetime alcohol use occasions | 1.52 | 2.87g | 69.01 | 110.58 | 4.99 | 135 | 0.000 | 0.863 | ||
Peak drinks on an occasion in past year | 0.46 | 0.96g | 9.30 | 4.60 | 15.41 | 135 | 0.000 | 2.66 | ||
Lifetime marijuana use occasions | 0.06 | 0.38h | 108.61 | 256.94 | 3.46 | 135 | 0.001 | 0.597 | ||
Reporting >30 lifetime marijuana use occasions (%) | 0 | 29 | ||||||||
Lifetime other drug use | 0.00 | 0.00 | 7.04 | 38.05 | 1.52 | 135 | 0.132 | 0.261 |
Measures
Substance use measures.
Demographic information.
Socioeconomic status.
Family background.
Pubertal development.
Psychopathology and mood.
Alcohol Expectancy Questionnaire–Adolescent version.
Neurocognition.
Continuous Nonusers (N=67) | Moderate to Heavy Alcohol Initiators (N=70) | ||||||||
---|---|---|---|---|---|---|---|---|---|
Variable | Domain Assessed | Mean | SD | Mean | SD | t | df | p | Cohen’s d |
Digit vigilance total time to complete (sec) | Sustained attention | 225.06 | 52.80a | 219.59 | 41.46a | 0.67 | 132 | 0.504 | 0.115 |
Wechsler Abbreviated Scale of Intelligence (WASI) block design raw score | Spatial perception, visual abstract processing, problem solving | 47.27 | 13.81a | 42.86 | 13.13a | 1.89 | 132 | 0.060 | 0.327 |
WASI matrix reasoning raw score | Nonverbal abstract problem solving, inductive reasoning | 27.80 | 3.62b | 26.41 | 3.34b | 2.31 | 131 | 0.023 | 0.399 |
WASI vocabulary raw score | Word knowledge, verbal concept formation | 53.02 | 7.09a | 51.96 | 7.06a | 0.87 | 132 | 0.389 | 0.150 |
WASI similarities raw score | Abstract verbal reasoning | 35.17 | 4.24a | 34.31 | 5.07a | 1.06 | 132 | 0.292 | 0.184 |
Delis-Kaplan Executive Function System (D-KEFS) trails number-letter switching time to complete (sec) | Flexibility of thinking on a visual-motor task | 70.60 | 19.45 | 77.46 | 28.75 | 1.63 | 135 | 0.106 | 0.279 |
D-KEFS towers total achievement score (raw) | Spatial planning, rule learning | 17.04 | 2.68 | 17.24 | 2.73 | 0.43 | 135 | 0.669 | 0.074 |
D-KEFS color word interference inhibition time to complete (sec) | Inhibitory functioning | 57.39 | 14.25 | 59.60 | 13.12 | 0.95 | 135 | 0.346 | 0.161 |
D-KEFS color word interference inhibition/switching time to complete (sec) | Inhibition/cognitive flexibility | 65.81 | 15.81 | 63.91 | 14.39 | 0.73 | 135 | 0.465 | 0.126 |
Rey-Osterrieth Complex Figure (ROCF) copy accuracy score | Visuospatial functioning; organization | 29.35 | 3.02a | 28.63 | 3.01a | 1.39 | 132 | 0.168 | 0.239 |
ROCF delay accuracy score | Spatial memory | 18.09 | 4.12c | 17.77 | 4.26c | 0.43 | 130 | 0.665 | 0.076 |
Wechsler Intelligence Scale for Children, 3rd edition (WISC-III), digits forward raw score | Attention | 9.91 | 1.84a | 10.17 | 1.94a | 0.81 | 132 | 0.421 | 0.138 |
WISC-III digits backward raw score | Short-term memory, attention | 6.44 | 1.74a | 6.19 | 2.05a | 0.76 | 132 | 0.447 | 0.131 |
WISC-III arithmetic raw score | Computational skills, auditory memory, attention, problem solving | 22.23 | 3.36a | 22.46 | 3.34a | 0.39 | 132 | 0.701 | 0.069 |
WISC-III coding raw score | Visual motor speed, visual memory, sustained effort and attention | 61.20 | 10.88a | 59.37 | 10.75a | 0.98 | 132 | 0.329 | 0.169 |
WISC-III mazes raw score | Planning, perceptual organization, visual-motor coordination and speed, nonverbal reasoning | 23.48 | 3.71a | 22.77 | 3.20a | 1.19 | 132 | 0.235 | 0.205 |
Wechsler Adult Intelligence Scale (WAIS-IV) letter-number sequence raw score | Attention, auditory short-term memory, sequencing ability | 10.69 | 1.94d | 10.09 | 1.88d | 1.65 | 108 | 0.103 | 0.314 |
Hooper Visual Organization Test total raw score | Visuospatial functioning and spatial integration | 25.32 | 2.26d | 24.94 | 2.40d | 0.86 | 108 | 0.392 | 0.163 |
California Verbal Learning Test (CVLT) list A total 1 to 5 raw score | Verbal learning and memory | 55.91 | 8.01a | 54.51 | 6.29a | 1.12 | 132 | 0.263 | 0.194 |
CVLT list A trial 1 raw score | Verbal learning and memory | 7.72 | 1.93a | 7.37 | 1.64a | 1.13 | 132 | 0.262 | 0.195 |
CVLT list A trial 5 raw score | Verbal learning and memory | 12.92 | 1.68a | 12.74 | 1.57a | 0.64 | 132 | 0.525 | 0.111 |
CVLT short delay free raw score | Verbal learning and memory | 11.87 | 2.07a | 11.74 | 2.29a | 0.33 | 132 | 0.743 | 0.060 |
CVLT short delay cued raw score | Verbal learning and memory | 12.15 | 1.94e | 12.19 | 1.70e | 0.12 | 129 | 0.907 | 0.022 |
CVLT long delay free raw score | Verbal learning and memory | 12.11 | 1.91f | 12.30 | 1.79f | 0.60 | 130 | 0.550 | 0.103 |
CVLT long delay cued raw score | Verbal learning and memory | 12.52 | 1.80f | 12.54 | 1.75f | 0.04 | 130 | 0.968 | 0.011 |
Wide Range Achievement Test–3 Reading raw score | Premorbid functioning and intellectual capacity | 45.44 | 4.14a | 44.67 | 4.04a | 1.08 | 132 | 0.280 | 0.188 |
Follow-up procedures.
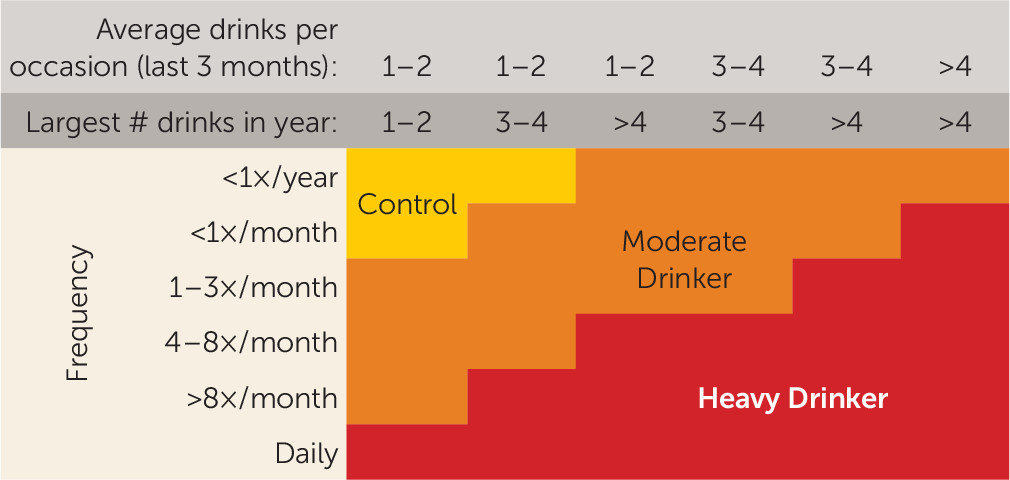
Procedures
Image acquisition.
Visual working memory task.
Data Analysis
Structural image processing.
Variable | Model 1 | Model 2 | Model 3 |
---|---|---|---|
Demographic and family variables | |||
1. Sex | X | X | X |
2. Baseline age | |||
3. Follow-up age | X | X | X |
4. Race | |||
5. Hollingshead Index of Social Position score (socioeconomic status) | X | X | X |
6. Family history density of alcohol or drug use disorder | |||
7. Pubertal Development Scale score | X | ||
8. Grade in school | |||
9. Birth order | |||
10. Living with both parents | |||
11. Parents’ marital status | |||
Youth behavior, mood, and cognition | |||
12. Dating status | X | X | X |
13. Child involvement in extracurricular activities | |||
14. Hours of video games per week | |||
15. Grade point average | X | ||
16. CBCL externalizing t score | |||
17. CBCL internalizing t score | |||
18. CBCL withdrawn t score | |||
19. CBCL somatic complaints t score | |||
20. CBCL anxious/depressed t score | |||
21. CBCL social problems t score | |||
22. CBCL thought problems t score | |||
23. CBCL attention problems t score | |||
24. CBCL delinquent behavior t score | |||
25. CBCL aggressive behavior t score | |||
26. CBCL total problem t score | X | ||
27. Conduct Disorders Questionnaire total score | X | X | X |
28. Beck Depression Inventory-II total score | |||
29. State-Trait Anxiety Inventory total score | |||
30. AEQ total score | X | X | X |
31. AEQ global positive score | X | X | X |
32. AEQ social behavior change score | X | X | X |
33. AEQ improved performance score | |||
34. AEQ sexual enhancement score | |||
35. AEQ impaired performance score | |||
36. AEQ increased arousal score | |||
37. AEQ relaxation total score | |||
38. CDDR baseline lifetime smoking days (<5 for all participants) | |||
39. CDDR baseline lifetime drinking days (<10) | |||
40. CDDR baseline lifetime marijuana use days (<3) | |||
41. Repetitions excluded from functional magnetic resonance imaging (fMRI) series due to motion | X | ||
Neuropsychological testing variables | |||
1. Digit vigilance total time to complete (sec) | X | X | |
2. WASI block design raw score | X | X | |
3. WASI matrix reasoning raw score | X | X | |
4. WASI vocabulary raw score | X | ||
5. WASI similarities raw score | |||
6. D-KEFS trails condition 4 (number-letter switching) time to complete (sec) | X | ||
7. D-KEFS towers total achievement raw score | |||
8. D-KEFS color word interference inhibition time to complete (sec) | |||
9. D-KEFS color word interference inhibition/switching time to complete (sec) | |||
10. ROCF copy accuracy score | |||
11. ROCF delay accuracy score | |||
12. WISC-III digits forward raw score | |||
13. WISC-III digits backward raw score | |||
14. WISC-III arithmetic raw score | |||
15. WISC-III coding raw score | |||
16. WISC-III mazes raw score | |||
17. WAIS-IV letter-number sequence raw score | |||
18. Hooper Visual Organization Test total raw score | |||
19. CVLT list A total 1 to 5 raw score | |||
20. CVLT list A trial 1 raw score | |||
21. CVLT list A trial 5 raw score | |||
22. CVLT short delay free raw score | |||
23. CVLT short delay cued raw score | |||
24. CVLT long delay free raw score | |||
25. CVLT long delay cued raw score | |||
26. WRAT–3 reading raw score | |||
Cortical thickness and BOLD regionsb | |||
1. Banks of superior temporal sulcus | L-CT | ||
2. Caudal anterior cingulate | R-BOLD | ||
3. Caudal middle frontal | |||
4. Cuneus | |||
5. Entorhinal | |||
6. Fusiform | |||
7. Inferior parietal | |||
8. Inferior temporal | |||
9. Isthmus cingulate | |||
10. Lateral occipital | L-CT | ||
11. Lateral orbitofrontal | |||
12. Lingual | L-CT | ||
13. Medial orbitofrontal | |||
14. Middle temporal | R-CT, L-BOLD | ||
15. Parahippocampal | |||
16. Paracentral | |||
17. Pars opercularis | |||
18. Pars orbitalis | R-CT | ||
19. Pars triangularis | |||
20. Pericalcarine | |||
21. Postcentral | |||
22. Posterior cingulate | R-BOLD | ||
23. Precentral | |||
24. Precuneus | R-CT, L-BOLD, R-BOLD | ||
25. Rostral anterior cingulate | L-CT | ||
26. Rostral middle frontal | R-CT | ||
27. Superior frontal | R-CT | ||
28. Superior parietal | L-CT, R-CT | ||
29. Superior temporal | R-BOLD | ||
30. Supramarginal | L-CT | ||
31. Frontal pole | R-CT, R-BOLD | ||
32. Temporal pole | R-CT | ||
33. Transverse temporal | L-CT | ||
34. Insula |
Functional image processing.
Volumetric and functional image alignment.
Statistical Analysis
Results
Demographic Model
Demographic and Neuropsychological Performance Model
Demographic, Neuropsychological Performance, and Neuroimaging Model
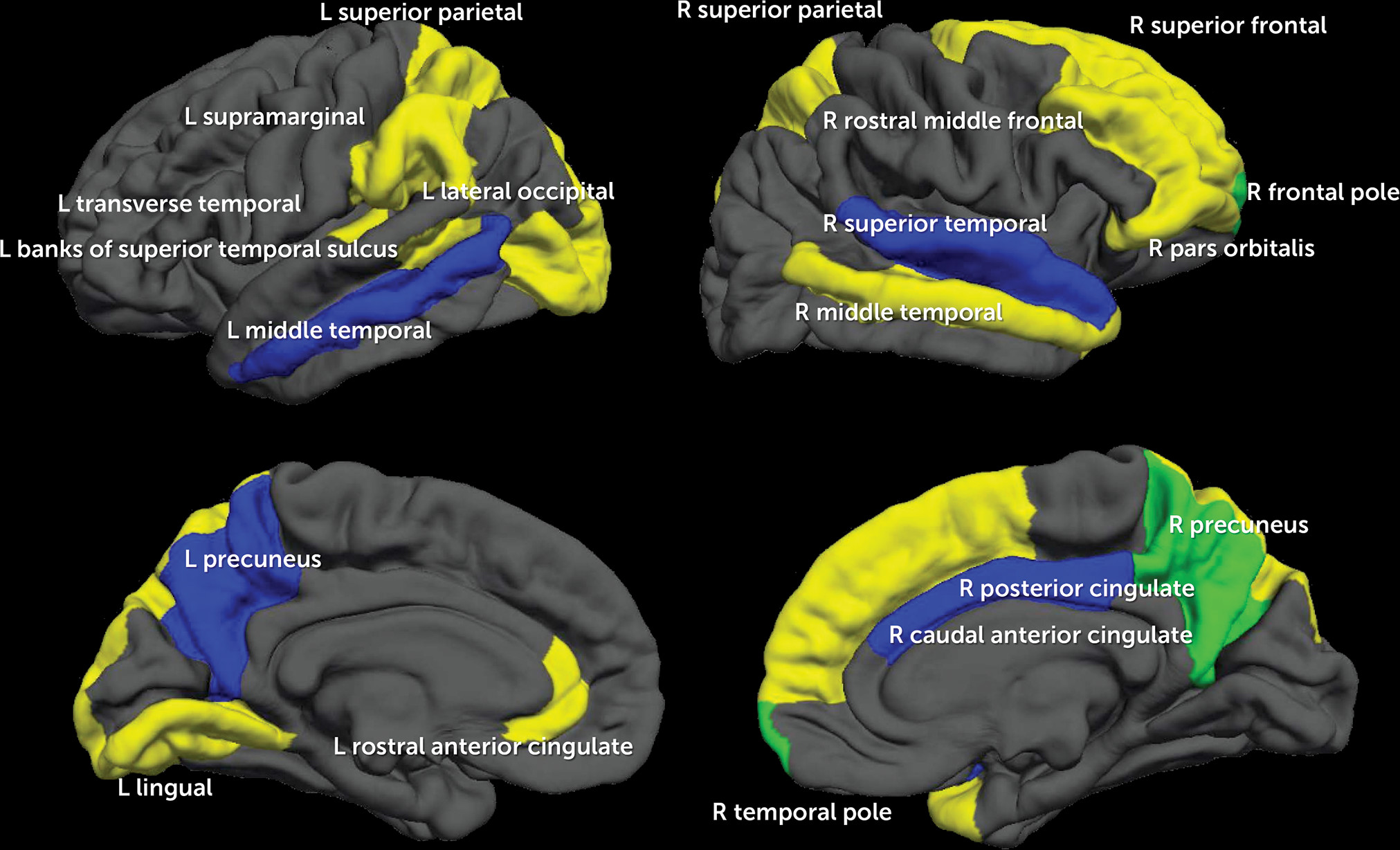
Further Investigation of Model 3
Measure | Continuous Nonusers (N=67) | Moderate to Heavy Alcohol Initiators (N=70) | p | Variable Importance | Cohen’s d | ||||
---|---|---|---|---|---|---|---|---|---|
Mean | SD | % | Mean | SD | % | ||||
1. L supramarginal cortical thickness | 2.96 | 0.14 | 2.89 | 0.13 | 0.002 | 10.01 | 0.518 | ||
2. Sex (female)b | 60 | 29 | >0.001 | 9.99 | 0.661 | ||||
3. R posterior cingulate BOLD | –0.63 | 3.90 | –2.19 | 3.60 | 0.016 | 8.84 | 0.416 | ||
4. R superior temporal BOLD | –1.17 | 3.60 | –1.89 | 2.83 | 0.194 | 8.45 | 0.222 | ||
5. Dating at baselineb | 22 | 61 | >0.001 | 8.08 | 0.860 | ||||
6. Socioeconomic status | 24.03 | 16.75 | 21.11 | 11.50 | 0.235 | 7.44 | 0.203 | ||
7. L transverse temporal cortical thickness | 2.78 | 0.21 | 2.68 | 0.21 | 0.006 | 7.37 | 0.476 | ||
8. R pars orbitalis cortical thickness | 3.02 | 0.22 | 2.92 | 0.19 | 0.005 | 7.28 | 0.487 | ||
9. WASI matrix reasoning raw score | 27.80 | 3.62 | 26.41 | 3.34 | 0.023 | 7.11 | 0.399 | ||
10. WASI block design raw score | 47.27 | 13.81 | 42.86 | 13.13 | 0.060 | 6.43 | 0.327 | ||
11. R rostral middle frontal cortical thickness | 2.53 | 0.10 | 2.49 | 0.11 | 0.058 | 6.06 | 0.381 | ||
12. L middle temporal BOLD | –1.11 | 2.56 | –1.62 | 2.47 | 0.238 | 5.83 | 0.203 | ||
13. R superior parietal cortical thickness | 2.60 | 0.12 | 2.55 | 0.10 | 0.016 | 5.76 | 0.453 | ||
14. L lingual cortical thickness | 2.26 | 0.16 | 2.28 | 0.13 | 0.367 | 5.62 | 0.137 | ||
15. R precuneus cortical thickness | 2.82 | 0.11 | 2.78 | 0.11 | 0.049 | 5.42 | 0.364 | ||
16. R caudal anterior cingulate BOLD | 2.40 | 4.90 | 0.43 | 4.66 | 0.017 | 5.24 | 0.412 | ||
17. AEQ social behavior change total scoreb | 36.92 | 7.41 | 41.23 | 7.94 | 0.001 | 5.23 | 0.561 | ||
18. R temporal pole cortical thickness | 3.88 | 0.30 | 3.76 | 0.37 | 0.041 | 5.18 | 0.356 | ||
19. R precuneus BOLD | –1.46 | 5.64 | –3.06 | 4.90 | 0.078 | 4.81 | 0.303 | ||
20. Repetitions excluded from functional magnetic resonance imaging series due to motion | 4.46 | 6.77 | 7.03 | 8.82 | 0.060 | 4.44 | 0.327 | ||
21. L lateral occipital cortical thickness | 2.45 | 0.16 | 2.46 | 0.14 | 0.774 | 4.41 | 0.067 | ||
22. Conduct Disorder Questionnaire total score | 0.45 | 1.08 | 1.17 | 1.88 | 0.007 | 4.04 | 0.470 | ||
23. R frontal pole BOLD | 2.02 | 4.74 | 0.50 | 7.54 | 0.161 | 3.91 | 0.241 | ||
24. R frontal pole cortical thickness | 3.10 | 0.28 | 2.99 | 0.33 | 0.038 | 3.80 | 0.360 | ||
25. L rostral anterior cingulate cortical thickness | 3.20 | 0.30 | 3.12 | 0.26 | 0.107 | 3.71 | 0.285 | ||
26. AEQ total score | 79.14 | 32.23 | 92.45 | 29.75 | 0.013 | 2.88 | 0.429 | ||
27. R middle temporal cortical thickness | 3.26 | 0.14 | 3.22 | 0.18 | 0.192 | 2.85 | 0.248 | ||
28. L banks superior temporal sulcus cortical thickness | 2.85 | 0.19 | 2.80 | 0.16 | 0.104 | 2.67 | 0.285 | ||
29. L precuneus BOLD | –2.03 | 5.85 | –3.46 | 5.17 | 0.130 | 2.63 | 0.260 | ||
30. L superior parietal cortical thickness | 2.60 | 0.12 | 2.5 | 0.11 | 0.015 | 2.55 | 0.869 | ||
31. AEQ global positive total score | 37.81 | 8.37 | 40.83 | 9.53 | 0.051 | 2.42 | 0.336 | ||
32. Digit vigilance completion time (seconds) | 225.06 | 52.80 | 219.59 | 41.46 | 0.504 | 2.36 | 0.115 | ||
33. Right superior frontal cortical thickness | 3.05 | 0.15 | 3.01 | 0.13 | 0.076 | 2.19 | 0.285 | ||
34. Follow-up age | 18.20 | 0.66 | 18.20 | 0.57 | 0.943 | 1.22 | 0.000 |
Discussion
Acknowledgments
Supplementary Material
- View/Download
- 162.34 KB
- View/Download
- 1002.08 KB
References
Information & Authors
Information
Published In
History
Keywords
Authors
Competing Interests
Funding Information
Metrics & Citations
Metrics
Citations
Export Citations
If you have the appropriate software installed, you can download article citation data to the citation manager of your choice. Simply select your manager software from the list below and click Download.
For more information or tips please see 'Downloading to a citation manager' in the Help menu.
View Options
View options
PDF/EPUB
View PDF/EPUBLogin options
Already a subscriber? Access your subscription through your login credentials or your institution for full access to this article.
Personal login Institutional Login Open Athens loginNot a subscriber?
PsychiatryOnline subscription options offer access to the DSM-5-TR® library, books, journals, CME, and patient resources. This all-in-one virtual library provides psychiatrists and mental health professionals with key resources for diagnosis, treatment, research, and professional development.
Need more help? PsychiatryOnline Customer Service may be reached by emailing [email protected] or by calling 800-368-5777 (in the U.S.) or 703-907-7322 (outside the U.S.).