Toward Systems Neuroscience of ADHD: A Meta-Analysis of 55 fMRI Studies
Abstract
Objective
Method
Results
Conclusions
Method
Search Strategy
Study Eligibility Criteria
Data Extraction
Meta-Analytic Technique
ADHDc | Comparison Subjectsd | |||||||||||||||
---|---|---|---|---|---|---|---|---|---|---|---|---|---|---|---|---|
Age | Age | |||||||||||||||
First Authorb | N | M | Mean | SD | N | M | Mean | SD | ADHD Type | Psychiatric Comorbidities | Treatment With Stimulants | Withdrawal From Stimulants | Task(s) | Contrast(s) | Number of Foci | Correction for Multiple Comparisonse |
Banich | 23 | 14 | 20 | 1.7 | 23 | 13 | 19 | 0.9 | Co | No | 20 lifetime, 14 current | 24 hours | Color word Stroop (variant) | Six contrasts included in the meta-analysisf | 20 (total) | Cluster-wise protection; voxel level threshold p=0.01 (AFNI AlphaSim) |
Bayerl | 30 | 16 | 31 | 0.6 | 30 | 16 | 31 | 8.4 | Co | No | All naive | N/A | 2-back working memory | C>A | 1 | Cluster-size thresholding (50 voxels); voxel level p=0.001 uncorrected (SPM99) |
Booth | 12 | 8 | 9.4 | 1.2 | 12 | 7 | 9.3 | 12 | 8 Co, 4 I | No | All current | 48 hours | Go/no-go selective attention | C>A; C>A | 17; 3 | Cluster-size thresholding (10 voxels); voxel level p<0.001 uncorrected (SPM99) |
Braet | 20 | 17 | 14 | 2.1 | 38 | 31 | 13 | 1.9 | NS | NS | Some (NS) current | 24 hours | Sustained Attention to Response Test (go/no-go) | C>A: successful inhibition | 5 | NS for second-level analysis (AFNI) |
Cao | 12 | 12 | 13 | 1.7 | 13 | 13 | 13 | 1.2 | 7 Co, 5 I | 5 ODD, 2 CD | 3 current | 2 weeks | Cued target detection | C>A: intrinsic alerting effect; C>A: phasic alerting effect; C>A: alerting effect | 3; 4; 3 | Cluster-size thresholding (10 voxels); voxel level p<0.001 uncorrected (SPM2) |
Cerullo | 10 | 7 | 14 | 2 | 13 | 7 | 15 | 1.9 | NS | 2 ODD, 2 tics | 8 current | Day of the scan | CPT-X | C>A; A>C | 1; 2 | Cluster-size thresholding (137 voxels); voxel-level p<0.05 uncorrected (AFNI) |
Cubillo (2010) | 11 | 11 | 29 | 1 | 14 | 14 | 28 | 2 | 6 Co, 2 HI, 3 I | 1 AD, 3 MD, 1 CD, 2 SUD, 1 ND | All naive | N/A | Tracking stop | C>A: successful stop-go trials; C>A: unsuccessful stop-go trials; C>A: switch task | 2; 1; 2 | Cluster-mass threshold; voxel-wide significance: p<0.05; cluster threshold p<0.01 (XBAM) |
Cubillo (2011) | 11 | 11 | 29 | 1 | 15 | 15 | 28 | 3 | 6 Co, 2 HI, 3 I | 1 AD, 3 MD, 1 CD, 2 SUD, 1 ND | All naive | N/A | Simon task | C>A: incongruent-congruent; C>A: oddball-congruent | 1; 1 | Cluster-mass threshold; voxel-wide significance: p<0.05; cluster threshold p<0.01 (XBAM) |
Cubillo (2012) | 11 | 11 | 29 | 1 | 15 | 15 | 28 | 3 | 6 Co, 2 HI, 3 I | 1 AD, 3 MD, 1 CD, 2 SUD, 1 ND | All naive | N/A | Rewarded CPT | C>A: effect of attention; C>A: effect of reward | 3; 2 | Cluster-mass threshold; voxel-wide significance: p<0.05; cluster threshold 0.01 (XBAM) |
Dibbets (2009) | 16 | 16 | 29 | 6.4 | 13 | 13 | 29 | 6.4 | All Co | No | 14 current | 24 hours | Modified go/no-go | A>C: overall activation | 1 | Cluster-size thresholding (3 voxels); voxel level p<0.05 (SPM2) |
Dibbets (2010) | 15 | 15 | 29 | 6.2 | 14 | 14 | 29 | 6.4 | All Co | No | 14 current | 24 hours | Switch | C>A; A>C | 6; 6 | Cluster-level thresholding (1,000 iterations; p<0.05) (Brainvoyager QX) |
Dillo | 15 | 11 | 21–42 | N/A | 15 | 11 | 21–46 | N/A | 5 Co, 7 I, 3 HI | No | Some (NS) current | 3 weeks | Go/no-go | A>C | 2 | N/S (SPM2) |
Durston (2003) | 7 | 6 | 8.5 | 1.6 | 7 | 6 | 8.7 | 1.5 | 4 Co, 3 I | ODD or CD (NS) | All current | 24 hours | Go/no-go | C>A: no-go > go; A>C: no-go > go | 1; 8 | Cluster-size thresholding (3 voxels); voxel-level p<0.05 (NeuroImaging Software) |
Durston (2007) sample 1 | 10 | 8 | 12 | 2.6 | 10 | 8 | 12 | 2.1 | 5 Co, 2 I, 3 HI | 4 ODD | 5 current | 24 hours | Variant go/no-go | C>A: unexpected stimulus-unexpected time | 1 | Cluster-size thresholding (5 voxels); voxel-level p<0.05 (SPM2) |
Durston (2007) sample 2 | 12 | 12 | 15 | 2.3 | 12 | 12 | 15 | 2.1 | 9 Co, 3 HI | 4 ODD | 9 current | 24 hours | Variant go/no-go | C>A: expected stimulus-unexpected time; C>A: unexpected stimulus-expected time | 1; 2 | Cluster-size thresholding (5 voxels); voxel-level p<0.05 (SPM2) |
Hale | 10 | 9 | 35 | 8.1 | 10 | 9 | 27 | 4.1 | 3 Co, 7 I | 1 GAD + SoP + SP, 1 SP | 4 current | 2 weeks | WAIS forward/ backward digit span | C>A: backward; A>C: forward; A>C: backward | 5; 7; 3 | Cluster-size thresholding (25 voxels); voxel level p<0.001 uncorrected (SPM2) |
Karch | 8 | 7 | 38 | 7.8 | 8 | 7 | 38 | 6.6 | All Co | No | All naive | N/A | Auditory go/no-go (modified) | C>A | 7 | Cluster-size thresholding; p<0.001 uncorrected; threshold: 10 voxels (BrainVoyager) |
Kobel (2009) | 14 | 14 | 10 | 1.3 | 12 | 12 | 11 | 1.6 | 9 Co, 5 I | 5 ODD-CD, 4 GAD | All current | 24 hours | n-back | C>A: averaged 2- and 3-back | 5 | Family-wise error correction (p<0.05); cluster size: 10 voxels (SPM5) |
Kobel (2010) | 14 | 14 | 10 | 1.3 | 12 | 12 | 11 | 1.6 | 9 Co, 5 I | 5 ODD-CD, 4 GAD | All current | No | n-back | C>A: activation in 3-back task | 1 | Correction applied but type of correction NS (SPM 5) |
Konrad | 16 | 16 | 10 | 1.9 | 16 | 16 | 10 | 1.3 | 9 Co, 6 I, 1 HI | 5 ODD, 3 AD | All naive | N/A | Attention Network Test (modified) | C>A: alerting; C>A: executive control; A>C: alerting; A>C: reorienting; A>C: executive control | 1; 2; 1; 3; 1 | Cluster-size thresholding (10 voxels); p<0.001 uncorrected (SPM2) |
Kooistra | 10 | 10 | 22 | NS | 10 | 10 | 22 | NS | NS | N/A | No after age 16 years | N/A | Go/no-go | C>A: go; A>C: go | 16; 2 | Corrected cluster significance threshold: p=0.01 (FSL) |
Krauel | 12 | 12 | 15 | 0.7 | 12 | 12 | 15 | 1.3 | 6 Co, 5 I, 1 HI | 3 ODD, 2 CD | 5 current | 24 hours | Recognition memory | C>A: neutral; A>C: emotional; A>C: neutral | 1; 4; 4 | Cluster-size thresholding (10 voxels); voxel-level p<0.001 uncorrected (SPM99) |
Mostofsky | 11 | 8 | 10 | 1.2 | 11 | 8 | 10 | 1.4 | 9 Co, 2 I | No | 8 current | 24 hours | Sequential finger tapping | C>A | 2 | Cluster-size thresholding (84 voxels); voxel-level p<0.001 uncorrected (SPM99) |
Passarotti (2010, J Intl Neuropsychol Soc) | 15 | 12 | 13 | 2.6 | 14 | 7 | 14 | 2.4 | All C | No | 5 current | Over a 3-week period | Emotional valence Stroop | C>A: negative > neutral; C>A: positive > neutral words; A>C: negative > neutral words; A>C: positive > neutral words | 2; 2; 4; 5 | Contiguity threshold: p<0.01 uncorrected; experiment-wise type 1: p <0.02 corrected (AFNI AlphaSim) |
Passarotti (2010, J Am Acad Child Adolesc Psychiatry) | 14 | 9 | 13 | 2.3 | 19 | 9 | 14 | 3.1 | All Co | No | NS | At least 4 days | Affective 2-back working memory | C>A: angry > neutral faces; A>C: angry > neutral faces; A>C: happy > neutral faces | 13; 1; 12 | Contiguity threshold: p<0.01 uncorrected; experiment-wise type 1: p<0.02 corrected (AFNI AlphaSim) |
Passarotti (2010, Psychiatry Res) | 11 | 6 | 13 | 2.7 | 15 | 7 | 14 | 3.1 | All Co | No | 6 current | 3 weeks | Response inhibition | C>A; A>C | 7; 3 | Contiguity threshold: p<0.01 uncorrected; experiment-wise type 1: p<0.02 corrected (AFNI AlphaSim) |
Prehen-Kristensen | 12 | NS | 13 | 1.8 | 12 | NS | 14 | 2 | 12 Co, 2 I | 5 ODD | All current | 48 hours | Delayed-match-to-sample paradigm | C>A | 15 | Cluster-size thresholding (5 voxels); voxel-level p<0.05 (SPM5) |
Rubia (1999) | 7 | 7 | 16 | NS | 9 | 9 | 15 | NS | All Co | CD (NS) | NS | 1 week | Stop; delay | C>A; C>A | 4; 2 | Permutation; voxel-wise probability type I error: 0.05 |
Rubia (2005) | 16 | 16 | 13 | 2.1 | 21 | 21 | 14 | 1.6 | All Co | 5 CD | All naive | N/A | Stop | C>A: successful > unsuccessful inhibition; C>A: unsuccessful inhibition > baseline go | 2; 2 | Cluster level difference; <1 false activated cluster at p<0.05 (voxel comparison); p<0.01 (cluster comparison) |
Rubia (2007) | 17 | 17 | 14 | 2 | 18 | 18 | 13 | 2 | All Co | No | All naive | N/A | Oddball | C>A: successful oddball > standard; C>A: standard > oddball | 3; 2 | Cluster level difference; <1 false activated cluster at p<0.05 (voxel comparison); p<0.01 (cluster comparison) |
Rubia (2008) | 20 | 20 | 13 | 1.5 | 20 | 20 | 14 | 2 | All Co | No | All naive | N/A | Tracking stop | C>A: successful > failed stop; C>A: failed stop > go; C>A: go > stop | 1; 1; 1 | Cluster level difference; <1 false activated cluster at p<0.05 (voxel comparison); p<0.03 (cluster comparison) |
Rubia (2009, Am J Psychiatry) | 18 | 18 | 13 | 1 | 16 | 16 | 13 | 3 | All Co | No | All naive | N/A | Rewarded CPT | C>A: effect of attention; C>A: effect of reward; A>C: effect of attention | 2; 1; 9 | Cluster level difference; <1 false activated cluster at p<0.05 (voxel comparison); p<0.01 (cluster comparison) |
Rubia (2009, J Child Psychol Psychiatry) | 20 | 20 | 13 | 1.4 | 20 | 20 | 14 | 1.9 | All Co | No | All naive | N/A | Simon | C>A: incongruent > oddball; C>A: oddball > congruent | 2; 2 | Cluster level difference; <1 false activated cluster at p<0.05 (both voxel and cluster comparison) |
Rubia (2009, Neuropsychopharmacology) | 13 | 13 | 13 | 1.4 | 13 | 13 | 13 | 1.8 | All Co | 1 CD | All naive | N/A | Rewarded CPT | C>A: attention; C>A: reward; A>C: reward | 13; 2; 4 | Cluster level difference; <1 false activated cluster at p<0.05 (voxel comparison); p<0.02 (cluster comparison) (XBAM) |
Rubia (2009, Philos Trans R Soc Lond B Biol Sci) | ||||||||||||||||
Study 1 | 10 | 10 | 14 | 2 | 10 | 10 | 15 | 4 | All Co | 1 CD | 4 naive, 6 current | 36 hours (N=6) | Delay discounting | C>A | 6 | Cluster level difference; <1 false activated cluster at p<0.05 (voxel comparison); p<0.006 (cluster comparison) |
Study 2 | 12 | 12 | 13 | 1 | 12 | 12 | 13 | 1 | All Co | 1 CD | All naive | N/A | Time discrimination | C>A; A>C | 1; 2 | Cluster level difference; <1 false activated cluster at p<0.05 (voxel comparison); p<0.006 (cluster comparison) |
Rubia (2010, Hum Brain Mapp, p 1823) | 14 | 14 | 13 | 1.1 | 20 | 20 | 14 | 1.9 | All Co | No | All naive | N/A | Switch | C>A | 2 | Cluster level difference; <1 false activated cluster at p<0.05 for both voxel and cluster comparison (XBAM) |
Rubia (2010, Hum Brain Mapp, p 287) | 18 | 18 | 14 | 1.1 | 20 | 20 | 15 | 1.1 | All Co | No | All naive | N/A | Visual tracking stop; Meiran switch | C>A: successful-stop; C>A: failed stop; C>A | 1; 2; 3 | Cluster level difference; <1 false activated cluster at p<0.05 (voxel comparison); p<0.003 (cluster comparison) (XBAM) |
Rubia (2011, Biol Psychiatry) | 12 | 12 | 13 | 1 | 13 | 13 | 13 | 1 | All Co | No | All naive | N/A | Visual tracking stop | C>A: successful inhibition; C>A: inhibition failure | 13; 13 | Threshold-free cluster enhancement (p<0.05) |
Rubia (2011, Neuropsychopharmacology) | 12 | 12 | 13 | 1 | 13 | 13 | 13 | 1 | All Co | No | All naive | N/A | Simon | C>A: Simon > oddball condition | 4 | Cluster level difference; <1 false activated cluster at p<0.05 (voxel comparison); p<0.01 (cluster comparison) (XBAM) |
Rubia (2011, Hum Brain Mapp) | 18 | 18 | 14 | 2 | 20 | 20 | 16 | 1 | All Co | No | All naive | N/A | Simon | C>A: oddball > congruent; C>A: incongruent > oddball | 2; 2 | Cluster level difference; <1 false activated cluster at p<0.05 (voxel comparison); p<0.01 (cluster comparison) (XBAM) |
Schulz | 5g | 5 | 18 | 1.3 | 5 | 5 | 18 | 1.4 | 1 Co, 3 I, 1 HI | 1 CD | 4 lifetimeh | N/A | Go/no-go | C>A: correct no-go > correct go; A>C: correct no-go > correct go | 2; 3 | Cluster-size thresholding (120 voxels); voxel level p<0.01 uncorrected (SPM99) |
Sheridan | 10 | 0 | 15 | 2 | 10 | 0 | 15 | 1.3 | 6 Co; 4 I | 2 ODD, 2 SP | 5 lifetimei, 2 current | 24 hours | Delay match-to-sample | C>A: activation at high load period | 2 | Gaussian field theory; p=0.05 corrected at cluster level (fMRIstat program) |
Silk (2008) | 12 | 12 | 11 | 1.5 | 12 | 12 | 11 | 1.5 | All Co | No | All naive | N/A | Raven’s Standard Progressive Matrices | C>A | 45 | Clusters of voxels (z>2.33) with cluster level p<0.05 corrected (FSL) |
Silk (2005) | 7 | 7 | 14 | 1.8 | 7 | 7 | 15 | 1.8 | All Co | No | All naive | N/A | Mental rotation | C>A; A>C | 8; 4 | Voxel level p<0.001 uncorrected; cluster level p<0.05 corrected |
Smith (2006) | ||||||||||||||||
Study 1 | 17 | 17 | 13 | 2 | 18 | 18 | 14 | 2 | All Co | 5 CD | All naive | N/A | Go/no-go | C>A: successful no-go stimuli | 1 | Cluster level difference; <1 false activated cluster at p<0.05 (voxel comparison); p<0.01 (cluster comparison) |
Study 2 | 14 | 14 | 13 | 1.8 | 27 | 27 | 14 | 1.8 | All Co | 5 CD | All naive | N/A | Switch | C>A: switch | 9 | Cluster level difference; <1 false activated cluster at p<0.05 (voxel comparison); p<0.01 (cluster comparison) |
Smith (2008) | 21 | 21 | 13 | 1.6 | 17 | 17 | 14 | 2.1 | All Co | 3 CD/ODD | All naive | N/A | Time discrimination | C>A: time discrimination > temporal order judgment | 2 | Cluster level difference; <1 false activated cluster at p<0.05 (voxel comparison); p<0.01 (cluster comparison) (XBAM) |
Spinelli (2011, J Am Acad Child Adolesc Psychiatry) | 13 | 9 | 11 | 1.4 | 17 | 8 | 11 | 1.2 | 10 Co, 3 I | 3 ODD, 1 SP | 2 current | 24 hours | Go/no-go | A>C: preerror versus precorrect inhibition | 2 | Spatial extent cluster size threshold to achieve a corrected statistical threshold of p=0.05 (SPM5) |
Spinelli (2011, J Child Psychol Psychiatry) | 13 | 9 | 11 | 1.4 | 17 | 8 | 11 | 1.2 | 10 Co, 3 I | 3 ODD, 1 SP | 2 current | 24 hours | Go/no-go | A>C: posterror versus postcorrect inhibition trials | 9 | Spatial extent cluster size threshold to achieve a corrected statistical threshold of p=0.05 (SPM5) |
Strohle | 10 | 10 | 32 | 8.1 | 10 | 10 | 32 | 9.9 | 4 Co, 4 I, 2 HI | No | All naive | N/A | Monetary Incentive Delay | C>A: anticipation of gain > nongain; A>C: outcome of gain > nongain | 1; 7 | p<0.05 false discovery rate-corrected (SPM2) |
Tamm (2004) | 10 | 10 | 16 | 1.4 | 12 | 12 | 16 | 0.8 | All Co | No | 5 current | 18 hours | Modified go/no-go | C>A: “A–B” contrast; A>C: “A–B” contrast | 1; 1 | Deactivation mask (z=1.67; p<0.05) (SPM99) |
Tamm (2006) | 14 | 14 | 14–18 | N/A | 12 | 12 | 14–18 | N/A | All Co | No | 5 current | 18 hours | Oddball | C>A: activation during the oddball event | 4 | NS |
Valera (2005) | 20 | 12 | 34 | 11.8 | 20 | 12 | 33 | 11.0 | NS | No | 10 lifetime | 24 hours | Variant of visual n-back test | C>A: 2-back minus X-task contrast | 2 | p=0.001 (uncorrected) with extent threshold determined by Gaussian random field theory (SPM99) |
Valera (2010, Biol Psychiatry) | 21 | 15 | 34 | 10.1 | 19 | 12 | 33 | 11.0 | 5 Co, 12 I | No | 9 current | 24 hours | Paced and unpaced finger tapping | C>A: paced finger tapping; C>A: unpaced finger tapping | 24; 17 | p<0.05, family-wise error corrected, with extent threshold of 20 contiguous voxels (SPM5) |
Valera (2010, Am J Psychiatry) | 44 | 23 | 37 | 11.0 | 49 | 23 | 33 | 10.0 | 13 Co, 17 I, 1 HI | No | 18 current | 24 hours | 2-back | C>A: 2-back visual memory task | 2 | p<0.05, corrected (Gaussian random field theory) (SPM2) |
Vance | 12 | 12 | 11 | 1.5 | 12 | 12 | 10 | 1.3 | All Co | No | All naive | N/A | Mental rotation | C>A | 4 | Cluster-level threshold p<0.05, corrected (FSL) |
Wolf | 13 | 13 | 22 | 4.4 | 12 | 12 | 22 | 4.7 | 9 Co, 2 I, 2 HI | No | All lifetime | 6 at least 6 weeks, 6 for 3 days | Cognitive activation | C>A: increasing working memory load | 5 | p<0.05, false discovery rate-corrected (SPM5) |
Activation Liklihood Estimation Results in Relation to Neuronal Networks
Results
Studies Included in the Meta-Analysis
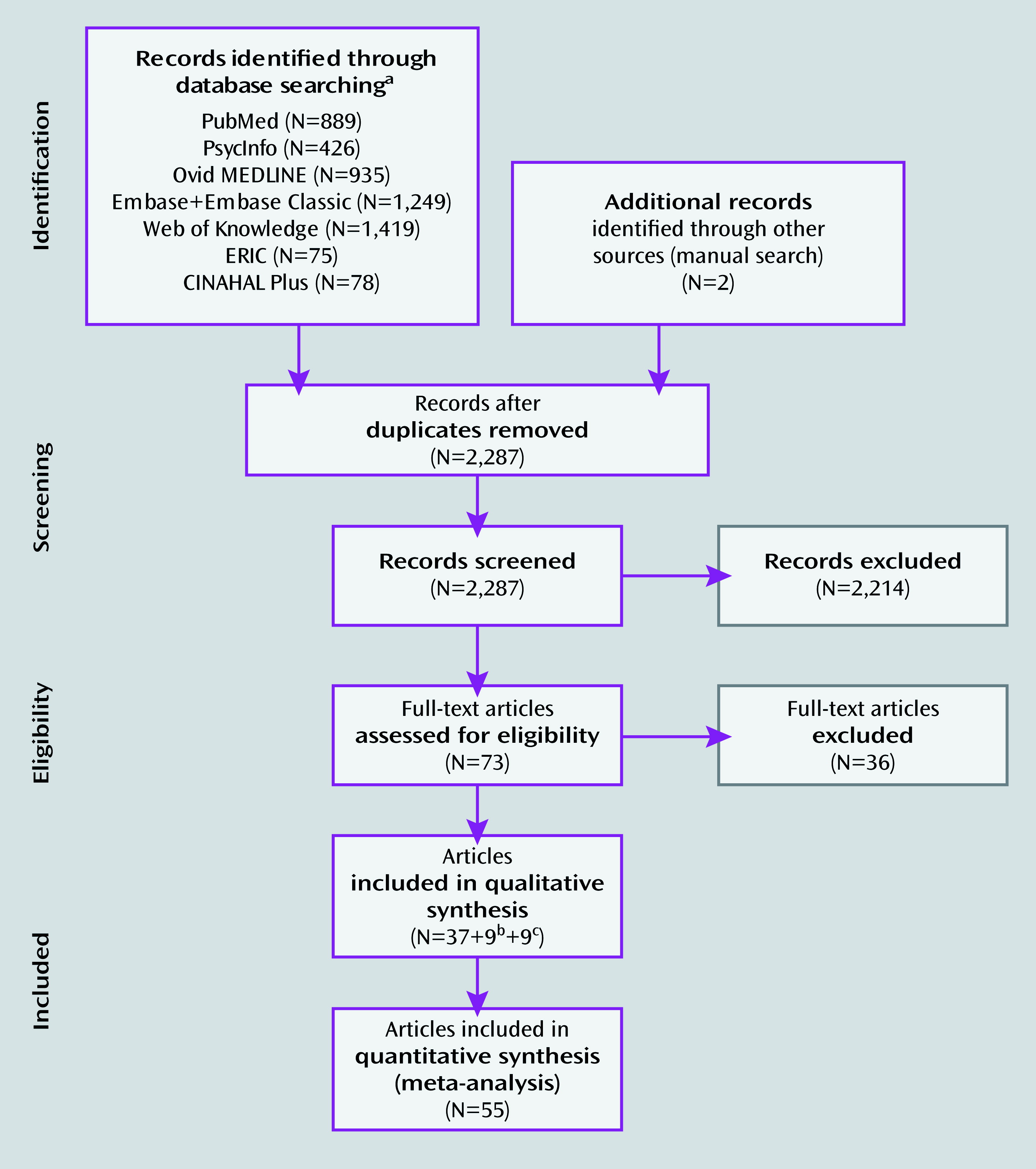
Activation Likelihood Estimation Results
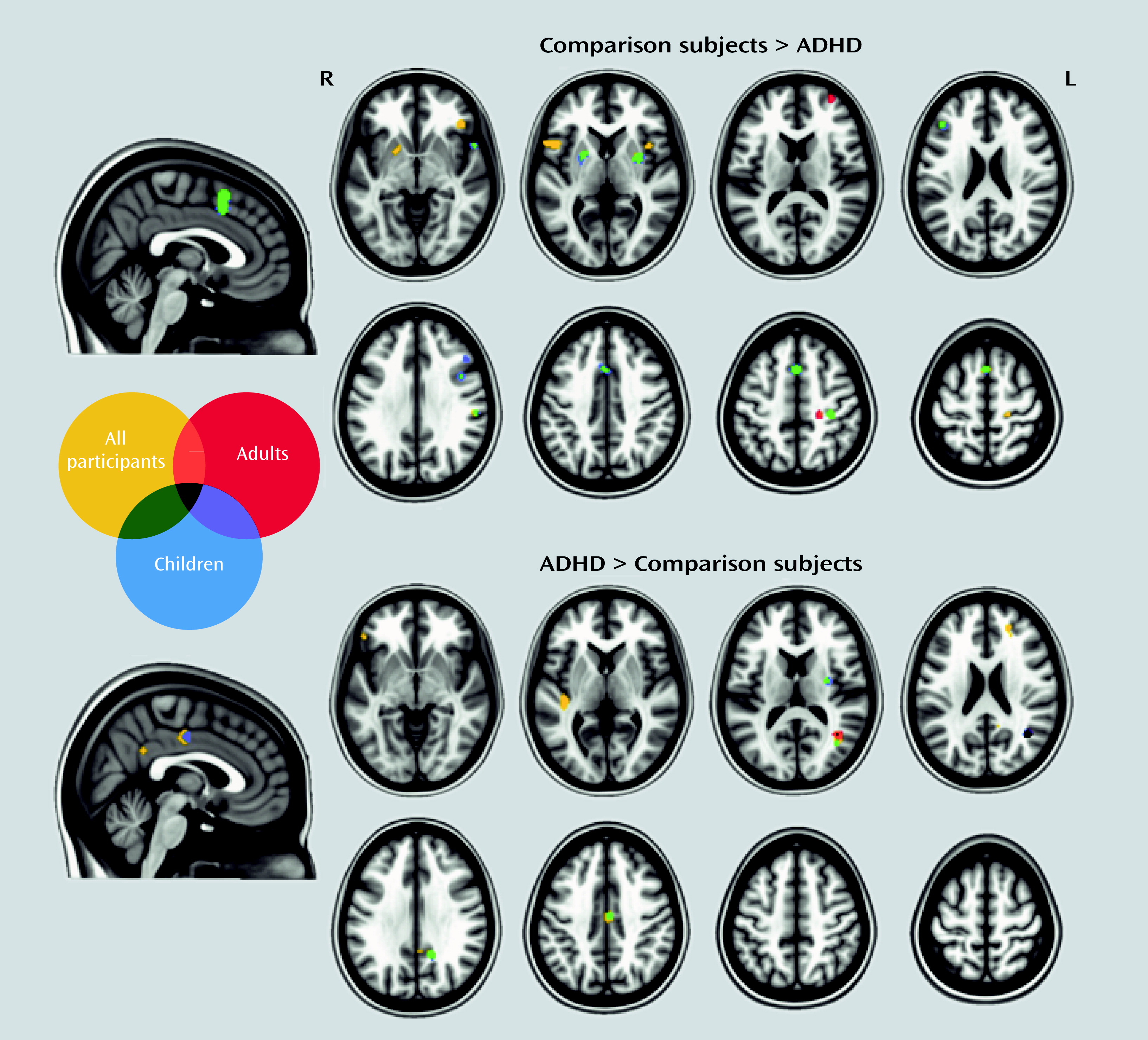
Weighted Centera | Maximum Activation Likelihood Estimate Valuea | ||||||||
---|---|---|---|---|---|---|---|---|---|
Contrast, Analysis, and Cluster Number | Volume (mm3) | x | y | z | Extrema Value | x | y | z | Anatomical Labelb |
Comparison subjects > participants with ADHD | |||||||||
Children, all tasksc | |||||||||
1 | 1,864 | −0.35 | 15.56 | 48.61 | 0.0211 | 0 | 16 | 54 | Medial superior frontal gyrus/supplementary motor area (BA 6) R, L (frontoparietal/ventral attention) |
0.0186 | 0 | 14 | 44 | Paracingulate gyrus (BA 32) R, L (ventral attention) | |||||
2 | 688 | 31.42 | 0.19 | 3.33 | 0.0148 | 32 | 0 | 4 | Putamen R |
3 | 464 | −21.92 | 2.37 | 4.1 | 0.0119 | −20 | 4 | 4 | Putamen L |
4 | 424 | 33.7 | −27.47 | 50.75 | 0.0136 | 34 | −28 | 52 | Postcentral gyrus R (somatomotor) |
5 | 400 | 42.23 | 9.52 | 29.36 | 0.0134 | 42 | 10 | 30 | Inferior frontal sulcus; inferior precentral sulcus R (frontoparietal) |
6 | 384 | 57.29 | −25.00 | 28.42 | 0.0145 | 58 | −26 | 28 | Inferior parietal lobule (supramarginal gyrus; BA 40) R (ventral attention) |
7 | 368 | 55.66 | 12.64 | −6.64 | 0.0136 | 56 | 12 | −6 | Superior temporal gyrus (BA 22) R (somatomotor) |
8 | 280 | −41.26 | 31.46 | 23.46 | 0.0131 | −42 | 32 | 24 | Middle frontal gyrus (BA 9/46) L (frontoparietal) |
9 | 248 | 47.46 | 25.85 | 31.84 | 0.0115 | 48 | 26 | 32 | Middle frontal gyrus (BA 9/46) R (frontoparietal) |
Adults, all tasksc | |||||||||
1 | 400 | 21.7 | −27.31 | 50.84 | 0.0106 | 22 | −28 | 50 | Central sulcus/precentral gyrus R |
2 | 232 | 34.38 | 56.96 | 13.62 | 0.0103 | 34 | 56 | 14 | Middle frontal gyrus (BA 10) R (frontoparietal) |
Participants with ADHD > comparison subjects | |||||||||
Children, all tasksd | |||||||||
1 | 680 | 39.72 | −58.51 | 18.51 | 0.0135 | 40 | −58 | 20 | Angular gyrus; middle occipital gyrus R |
0.0079 | 40 | −66 | 14 | Angular gyrus; middle occipital gyrus R (visual) | |||||
2 | 400 | 15.02 | −50.94 | 31.01 | 0.0101 | 16 | −52 | 32 | Posterior cingulate cortex; subparietal sulcus R |
3 | 392 | 31.22 | −7.23 | 16.7 | 0.0107 | 32 | −8 | 16 | White matter R (suboperculum) |
4 | 352 | 4.67 | −13.72 | 42.61 | 0.0101 | 4 | −14 | 42 | Midcingulate cortex R (ventral attention) |
Adults, all tasksd | |||||||||
1 | 872 | 40.39 | −58.08 | 16.91 | 0.0152 | 40 | −58 | 18 | Angular gyrus; middle occipital gyrus R |
Results in Relation to Neuronal Networks
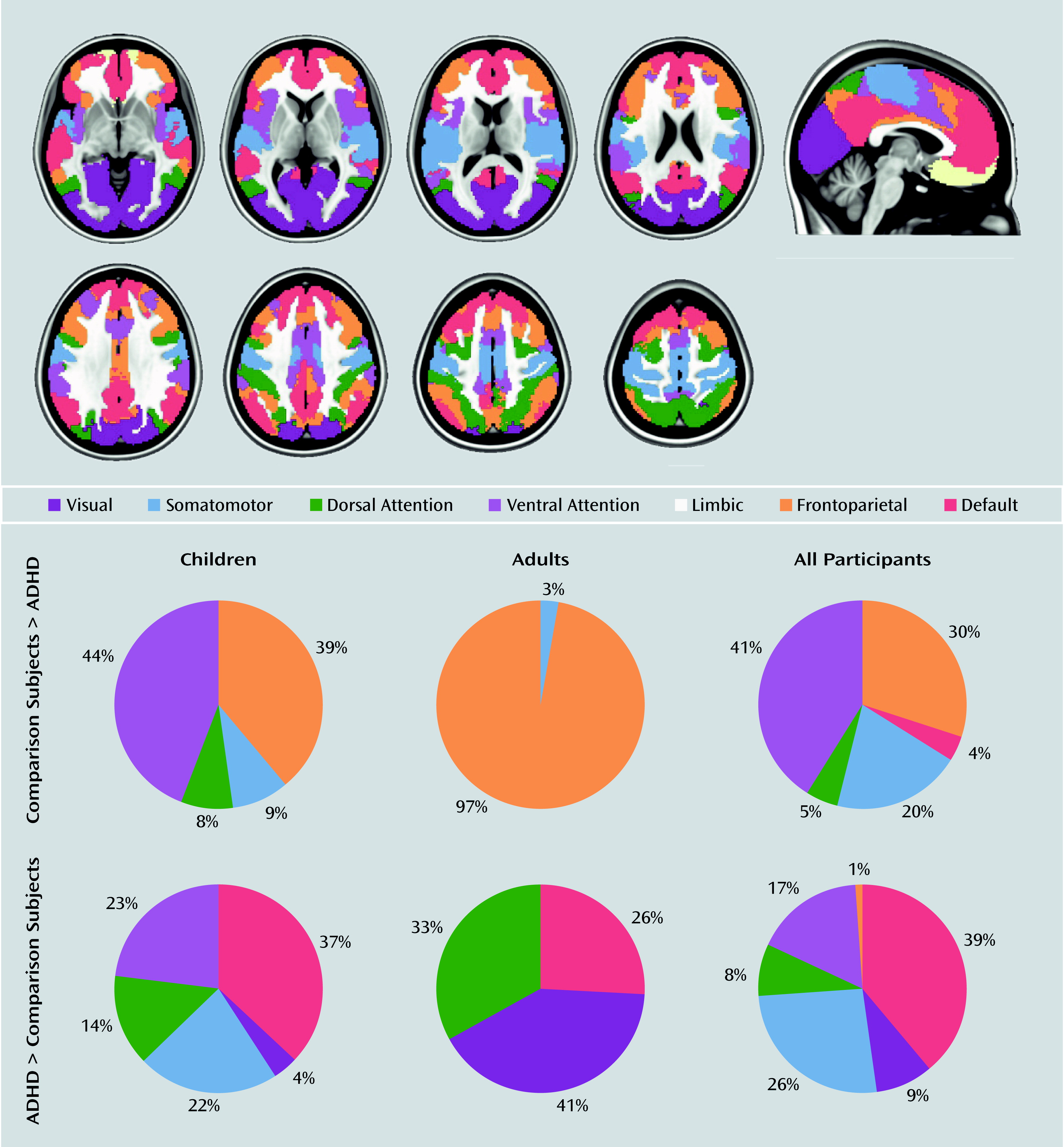
Discussion
Meta-Analyses
Children.
Adults.
Stimulant-naive individuals.
Comorbidity-free individuals.
Specific tasks.
Omnibus meta-analysis.
Subtraction analyses.
General Overview
Limitations
Conclusions
Supplementary Material
- View/Download
- 532.70 KB
References
Information & Authors
Information
Published In
History
Authors
Funding Information
Metrics & Citations
Metrics
Citations
Export Citations
If you have the appropriate software installed, you can download article citation data to the citation manager of your choice. Simply select your manager software from the list below and click Download.
For more information or tips please see 'Downloading to a citation manager' in the Help menu.
View Options
View options
PDF/EPUB
View PDF/EPUBGet Access
Login options
Already a subscriber? Access your subscription through your login credentials or your institution for full access to this article.
Personal login Institutional Login Open Athens loginNot a subscriber?
PsychiatryOnline subscription options offer access to the DSM-5-TR® library, books, journals, CME, and patient resources. This all-in-one virtual library provides psychiatrists and mental health professionals with key resources for diagnosis, treatment, research, and professional development.
Need more help? PsychiatryOnline Customer Service may be reached by emailing [email protected] or by calling 800-368-5777 (in the U.S.) or 703-907-7322 (outside the U.S.).