Genes, Brains, and Behavior: Imaging Genetics for Neuropsychiatric Disorders
Abstract
The Phenotype Bottleneck and Endophenotypes
Birth of Imaging Genetics: Candidate Gene Approach
Reference | Type of association | Target gene/SNP | Target phenotype | Sample size | Related disease | Findings |
---|---|---|---|---|---|---|
Egan et al. 2001100 | Candidate gene-candidate phenotype | rs4680 (COMT-Val158Met) | Frontal lobe activity during during executive cognition and memory task measured by fMRI | 175 patients (SZ), 219 unaffected siblings, and 55 controls | SZ | The Val allele is associated with a reduction in performance compared with the Met allele in three cohorts. |
Esslinger et al. 200952 | Candidate gene-candidate phenotype | rs134470 (ZNF804) | Connectivity between DLPFC and Hippocampus during memory task measured by fMRI | 115 healthy subjects | SZ, BD | GWAS supported risk variant rs1344706 impacted specifically on the connectivity between DLPFC and HF, in parallel with data found in subjects with SZ. |
Kohli et al. 201153 | Candidate gene-candidate phenotype | rs1545843 (SLC6A15) | Hippocampal volume measured by (1H-NMR) spectroscopy MRI | 80 patients with recurrent unipolar depression and 81control subjects. | MDD | rs1545843 showed associations with alterations in hippocampal volume and neuronal integrity. |
Flippini et al. 200954 | Candidate gene-brain-wide phenotype | rs 429358 (ApoE epsilon4) | Cortical atrophy across the brain assessed by VBM | 83 AD patients | AD | Gray matter volume decreased additively with increasing allele load in the medial and anterior temporal lobes bilaterally. |
Braskie et al. 201155 | Candidate gene-brain-wide phenotype | rs11136000 (CLU) | White matter integrity is determined by DTI | 398 healthy subjects | AD | Risk variant was associated with lower fractional anisotropy—a widely accepted measure of white matter integrity. |
Bis et al. 201240 | GWAS-candidate phenotype | GWAS: >3.1 million SNPs | Hippocampal volume measured by MRI | 9,232 healthy subjects | AD | The study identified two replicated hits rs17178006 and rs7294919 on chromosome 12 that correlate with hippocampal volume. |
Shen et. al. 201068 | GWAS × brain-wide phenotype | GWAS: 530,992 SNPs | Global gray matter density determined by VBM | 733 subjects (175 AD, 354 MCI, and 204 healthy controls, HC). | MCI, AD | SNPs in the APOE and TOMM40 genes were confirmed as markers strongly associated with multiple brain regions. Other top SNPs were proximal to the EPHA4, TP63 and NXPH1 genes. |
Hibar et al. 201170 | Multivariate GWAS × brain-wide phenotype | GWAS: 18,044 genes | 31,662 voxels of the whole brain- Structural MRI scans were analyzed by TBM | 202AD patients, 413 MCI, 237 Healthy elderly subjects | AD | Several known candidates were reidentified, as were other genes highly relevant to brain function. GAB2, which has been previously associated with late-onset AD, was identified as the top gene. |
Ge et al. 201271 | Multivariate GWAS × brain-wide phenotype | GWAS: 448,294 SNPs, 18,043 genes | 31,662 voxels of the entire brain MRI scans analyzed by TBM | 173 AD patients,361 MCI, 206 Healthy elderly subjects | AD | GRIN2B, which encodes the N-methyl-d-aspartate glutamate receptor NR2B subunit and affects both the parietal and temporal lobes in human brains. |
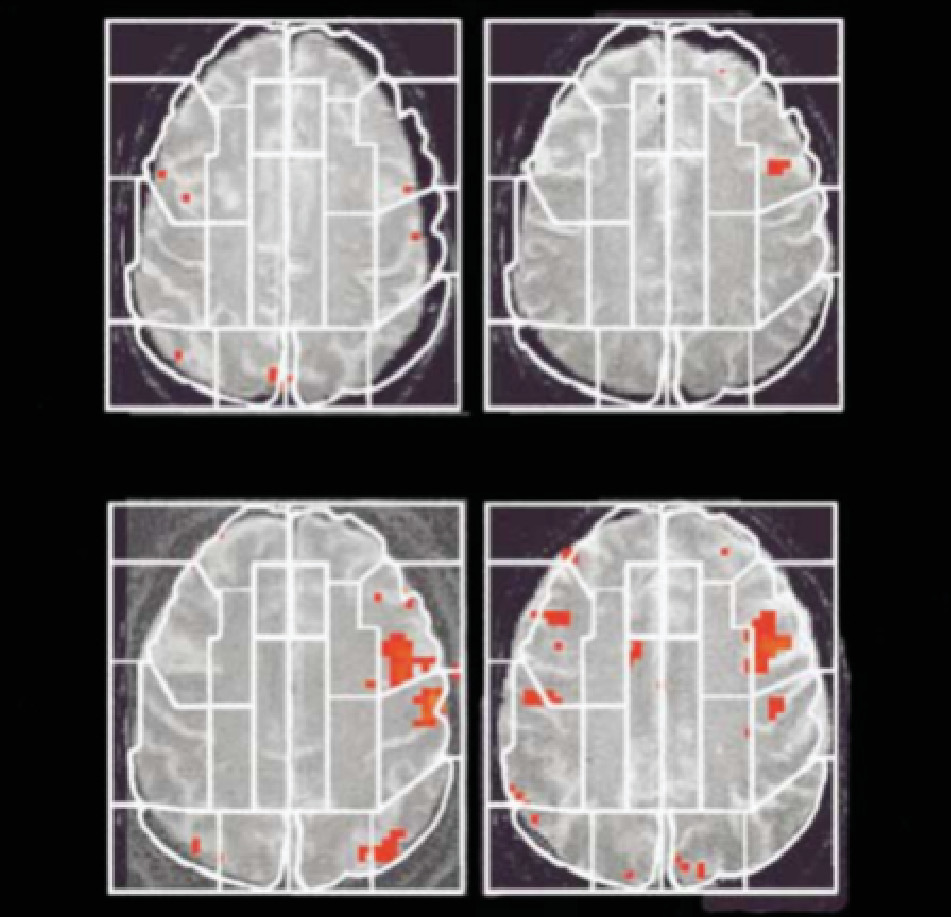
Stronger Candidates Supported by GWAS
Noncandidate Gene Approach
Genome-Wide Analysis Matched With Brain-Wide Scan
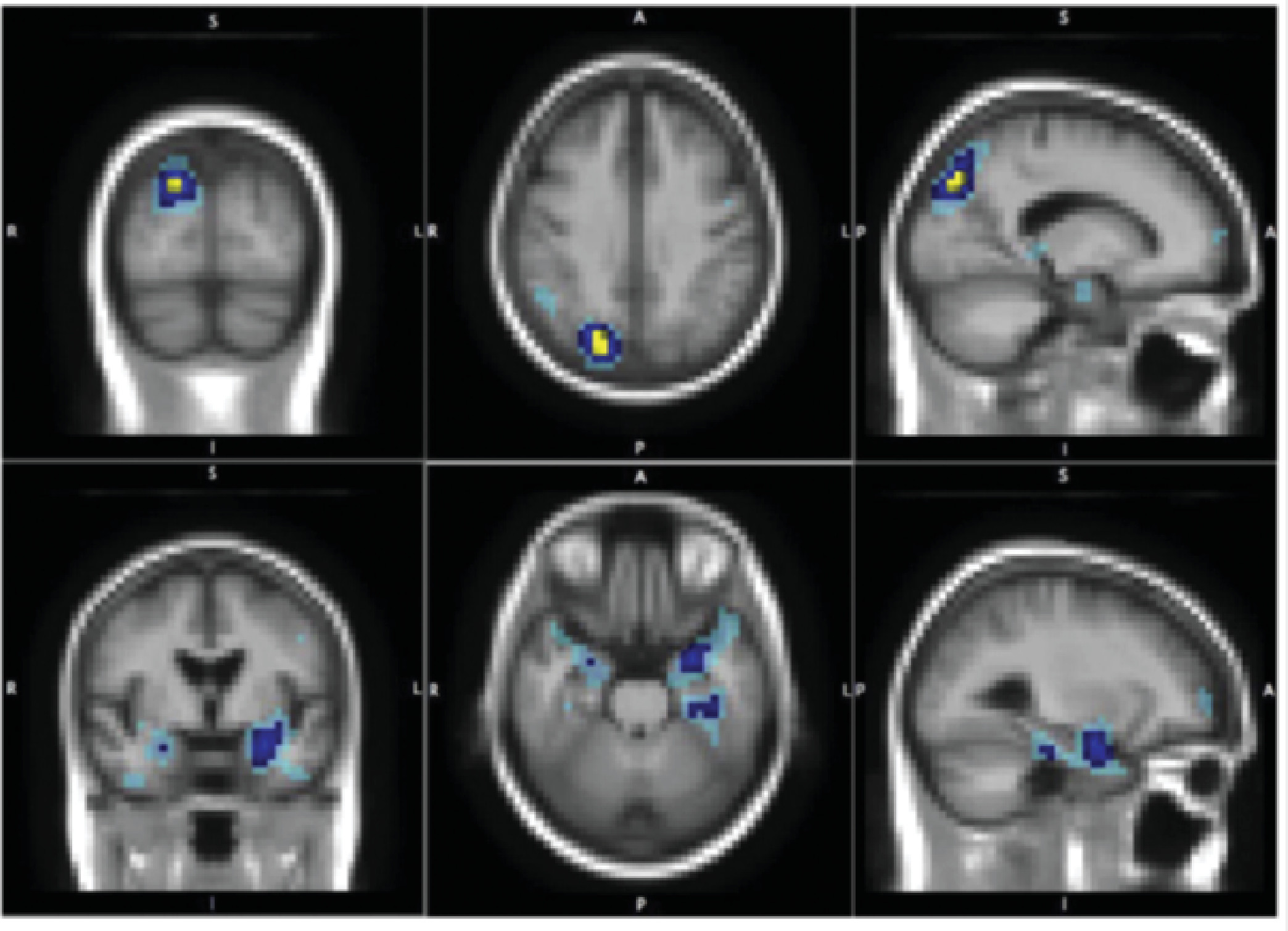
Gene–Environment Interactions
Conclusions
Acknowledgments
References
Information & Authors
Information
Published In
History
Authors
Metrics & Citations
Metrics
Citations
Export Citations
If you have the appropriate software installed, you can download article citation data to the citation manager of your choice. Simply select your manager software from the list below and click Download.
For more information or tips please see 'Downloading to a citation manager' in the Help menu.
View Options
View options
PDF/EPUB
View PDF/EPUBGet Access
Login options
Already a subscriber? Access your subscription through your login credentials or your institution for full access to this article.
Personal login Institutional Login Open Athens loginNot a subscriber?
PsychiatryOnline subscription options offer access to the DSM-5-TR® library, books, journals, CME, and patient resources. This all-in-one virtual library provides psychiatrists and mental health professionals with key resources for diagnosis, treatment, research, and professional development.
Need more help? PsychiatryOnline Customer Service may be reached by emailing [email protected] or by calling 800-368-5777 (in the U.S.) or 703-907-7322 (outside the U.S.).