Systematic Review of an Emerging Trend in China: Resting-State Functional Connectivity in Major Depressive Disorder
Abstract
Data Analysis | MDD>HC | MDD<HC | Reference |
---|---|---|---|
First-episode, treatment-naïve depressed subjects | |||
ReHo | Left middle temporal gyrus genotype- dependent | 92 | |
ReHo | No differences | No differences | 93 |
Seed-based anterior cingulate cortex and thalamus | Left parahippocampus, parietal lobe and frontal lobe | Right parietal lobe and cingulate gyrus | 45 |
Seed-based left posterior cingulate cortex | Bilateral dorsolateral prefrontal cortex and inferior parietal lobe | Medial prefrontal cortex / orbitofrontal cortex | 46 |
VMHC | Medial prefrontal cortex and posterior cingulate cortex / precuneus | 47 | |
ALFF | Fusiform gyri and anterior and posterior lobes of the cerebellum | Left inferior temporal gyrus and right inferior parietal lobe | 1 |
ALFF | Left superior occipital gyrus and cuneus | Right cerebellum posterior lobe, left parahippocampal gyrus and right middle frontal gyrus | 39 |
ALFF | Right dorsolateral frontal cortex, bilateral inferior frontal gyrus and orbital frontal region | Left insula, bilateral caudate and left hippocampus | 43 |
Network analysis | Left hippocampus and right parahippocampal gyrus | Left superior orbitofrontal cortex and left medial frontal cortex | 48 |
Graph analysis | Anterior cingulate cortex, dorsolateral, medial and inferior prefrontal cortex, insula, amgydala and superior and middle temporal gyri | 44 | |
Depressed outpatients | |||
ReHo | Insula, cerebellum anterior lobe, middle frontal gyrus, superior temporal gyrus, lentiform nucleus, precentral gyrus and postcentral gyrus | Interior frontal gyrus, inferior parietal lobule and posterior lobe of the cerebellum | 50 |
ReHo and ROI analysis | Bilateral anterior cingulate cortex, medial prefrontal cortex, right insula and right parahippocampalgyrus | Left posterior fusiform gyrus, inferior frontal area, inferior parietal lobule and caudate | 51 |
ReHo | Right orbitofrontal cortex, fusiform gyrus, ventral anterior cingulate gyrus, posterior cingulate gyrus. Left dorsal anterior cingulated gyrus and lentiform nucleus | 55 | |
ReHo | Right inferior temporal gyrus | Left posterior lobe of the cerebellum, right fusiform gyrus, left parahippocampal gyrus and right postcentral gyrus | 56 |
ReHo | Left thalamus, left temporal lobe, bilateral occipital lobe and left posterior lobe of the cerebellum | 94 | |
Seed-based cerebellar regions | Regions of the cerebellum, left middle frontal cortex and poles of the temporal cortex | Regions of the cerebellum, posterior cingulate cortex, ventromedial prefrontal cortex, superior frontal gyri, left orbitalfrontal gyrus and hippocampus | 12 |
Seed-based right dorsolateral prefrontal cortex | Right dorsolateral prefrontal cortex, left anterior cingulate cortex, left parahippocampal gyrus, thalamus and precentral gyrus | Right dorsolateral prefrontal cortex and right parietal lobe | 52 |
Seed-based left and right hippocampus | Left and right hippocampus, middle frontal gyrus, inferior parietal lobe and cerebellum | 53 | |
Seed-based left and right amygdala | Amygdala and left ventral prefrontal cortex | 54 | |
Seed-based 16 ROIs | Left thalamus and right cingulate cortex; right insula and precuneus | 57 | |
ALFF | Right dorsomedial frontal gyrus and right precuneus | Bilateral lingual gyri | 49 |
Treatment-resistant, depressed subjects | |||
ReHo | Left superior temporal gyrus, anterior and posterior lobe of the cerebellum, right cerebellar tonsil and bilateral fusiform gyrus | Left insula, superior temporal gyrus, inferior frontal gyrus, lingual gyrus and anterior lobe of the cerebellum | 95 |
Seed-based 16 ROIs | Precuneus, left middle frontal gyrus and cingulate cortex | 57 | |
Seed-based gray matter volume of right middle temporal gyrus and right caudate nucleus | Right precuneus, middle temporal gyrus, bilateral superior frontal gyrus and left middle frontal gyrus | Right cuneus | 63 |
Elderly depressed subjects | |||
ReHo | Left anterior insula, right posterior insula and right dorsolateral prefrontal cortex | Right orbitofrontal cortex, left middle frontal gyrus, left dorsolateral prefrontal, left inferior temporal gyrus and left postcentral gyrus | 67 |
ReHo | Superior frontal gyrus, postcentral gyrus and putamen | Superior and middle frontal and temporal gyri, postcentral gyrus, fusiform gyrus and precuneus | 68 |
Cohe-ReHo | Left posterior lobe of the cerebellum, left superior temporal gyrus, bilateral supplementary motor area and right postcentral gyrus | Left caudate nucleus, right anterior cingulate gyrus, left dorsolateral prefrontal cortex, right angular gyrus, bilateral medial prefrontal cortex and right precuneus | 64 |
Seed-based posterior cingulate cortex | Posterior cingulate cortex and temporal cortex | Posterior cingulate cortex and frontal cortex | 65 |
Suicidal, traumatized, or somatically ill depressed subjects | |||
ALFF | Parahippocampal gyrus, anterior cingular gyrus and angular gyrus of the parietal lobe | 69 | |
ALFF | Anterior and posterior lobes of the cerebellum | Right dorsolateral prefrontal cortex, ventromedial prefrontal cortex, superior frontal cortex, right middle temporal gyrus and rostral anterior cingulate cortex | 71 |
Graph analysis | Prefrontal cortex and anterior cingulate gyrus | 70 |
Methods
Literature Search
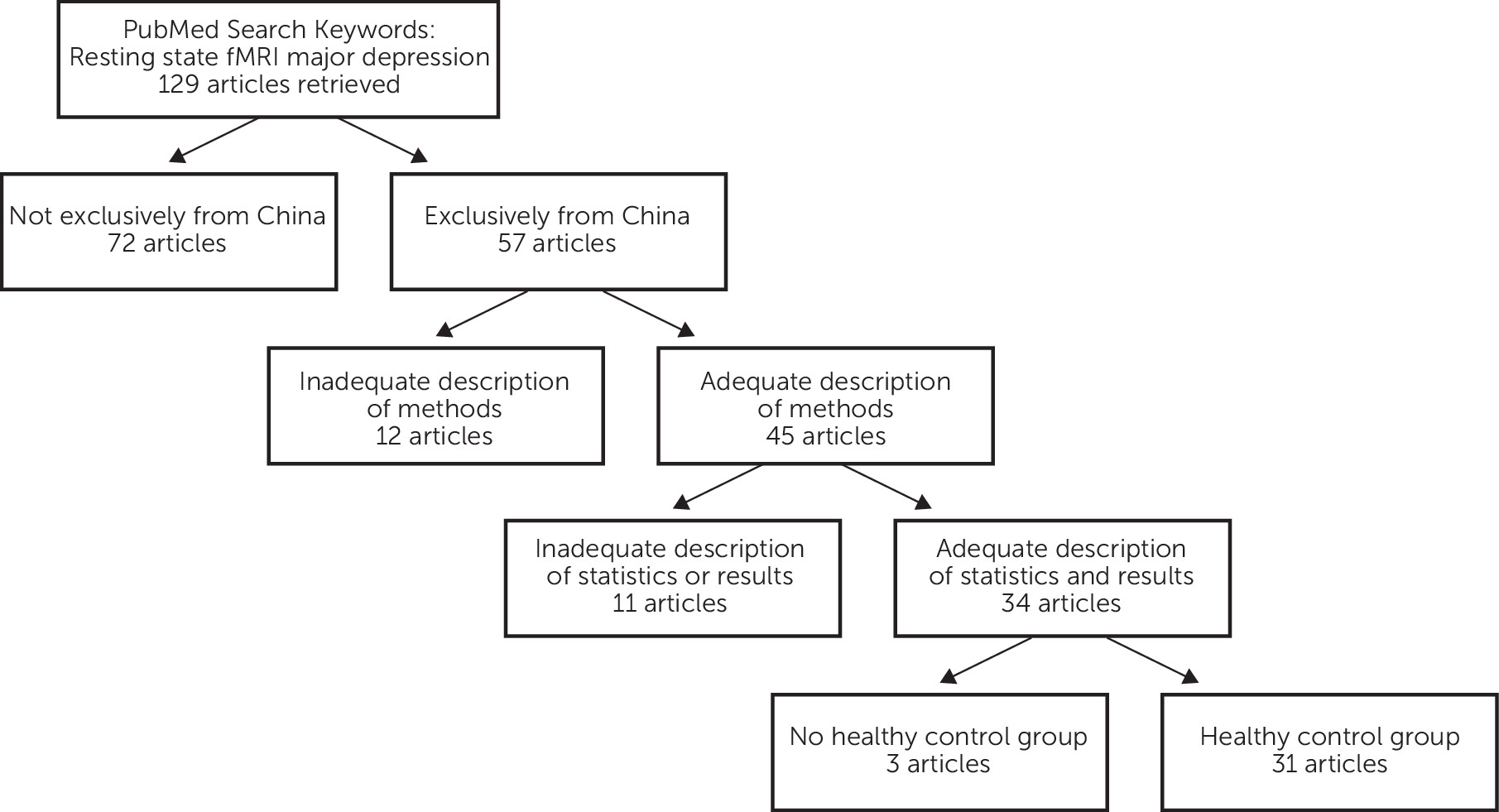
Main Features of Resting-State Neuroimaging
Main Features of Resting-State Functional Connectivity MRI
Main Principles of rs-fcMRI Data Analysis
Results
First-Episode, Treatment-Naïve Depressed Subjects
Depressed Outpatients
Treatment-Resistant Depressed Subjects
Elderly Depressed Subjects
Suicidal, Traumatized, or Somatically Ill Depressed Subjects
Discussion
Acknowledgments
References
Information & Authors
Information
Published In
History
Authors
Metrics & Citations
Metrics
Citations
Export Citations
If you have the appropriate software installed, you can download article citation data to the citation manager of your choice. Simply select your manager software from the list below and click Download.
For more information or tips please see 'Downloading to a citation manager' in the Help menu.
View Options
View options
PDF/EPUB
View PDF/EPUBGet Access
Login options
Already a subscriber? Access your subscription through your login credentials or your institution for full access to this article.
Personal login Institutional Login Open Athens loginNot a subscriber?
PsychiatryOnline subscription options offer access to the DSM-5-TR® library, books, journals, CME, and patient resources. This all-in-one virtual library provides psychiatrists and mental health professionals with key resources for diagnosis, treatment, research, and professional development.
Need more help? PsychiatryOnline Customer Service may be reached by emailing [email protected] or by calling 800-368-5777 (in the U.S.) or 703-907-7322 (outside the U.S.).