Minds and Brains, Sleep and Psychiatry
Abstract
Objective
Methods
Results
Conclusions
HIGHLIGHTS
Philosophy and Physiology
Neurophysiology
Lucid Dreaming
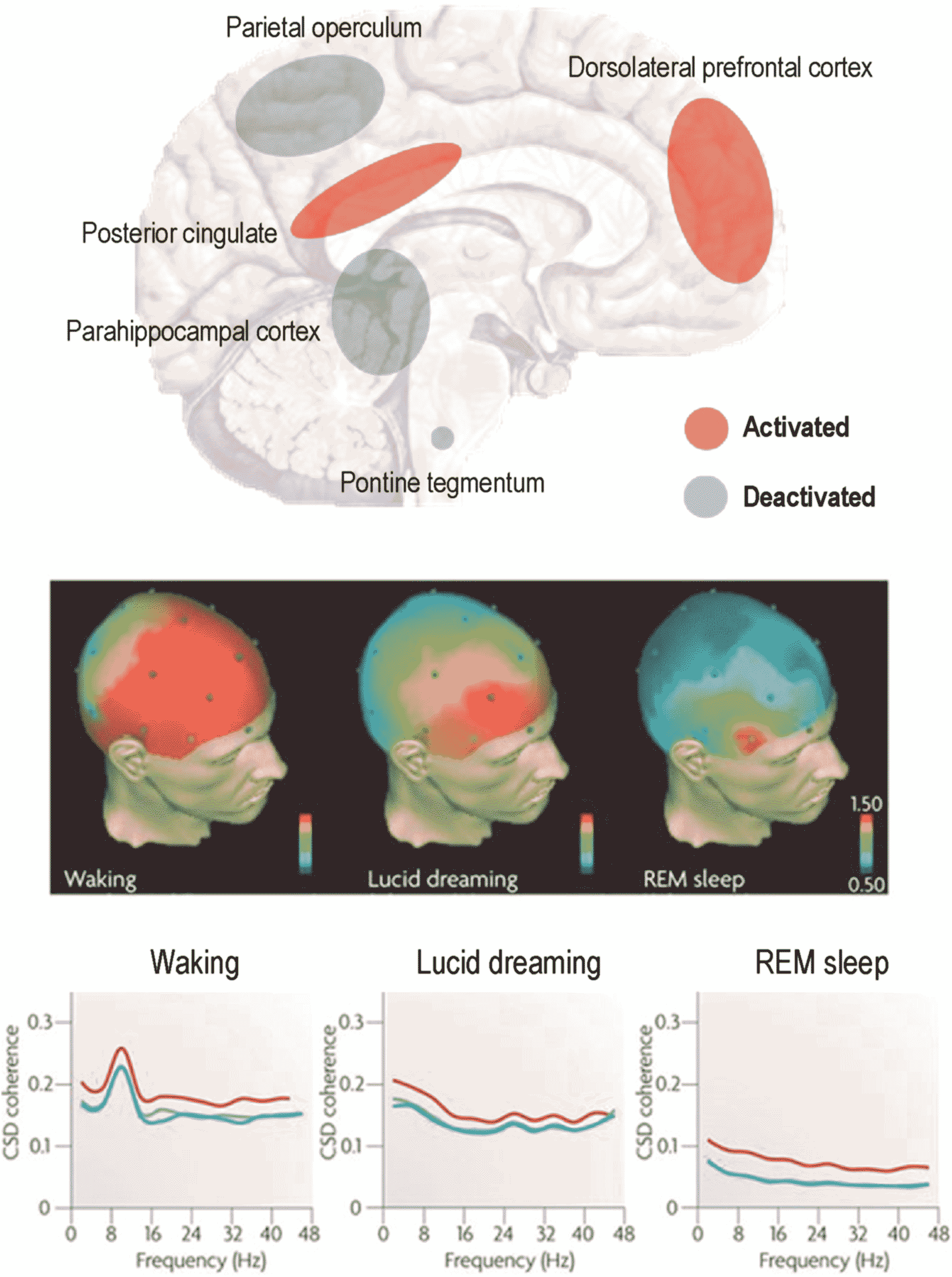
Human REM Sleep Signaling
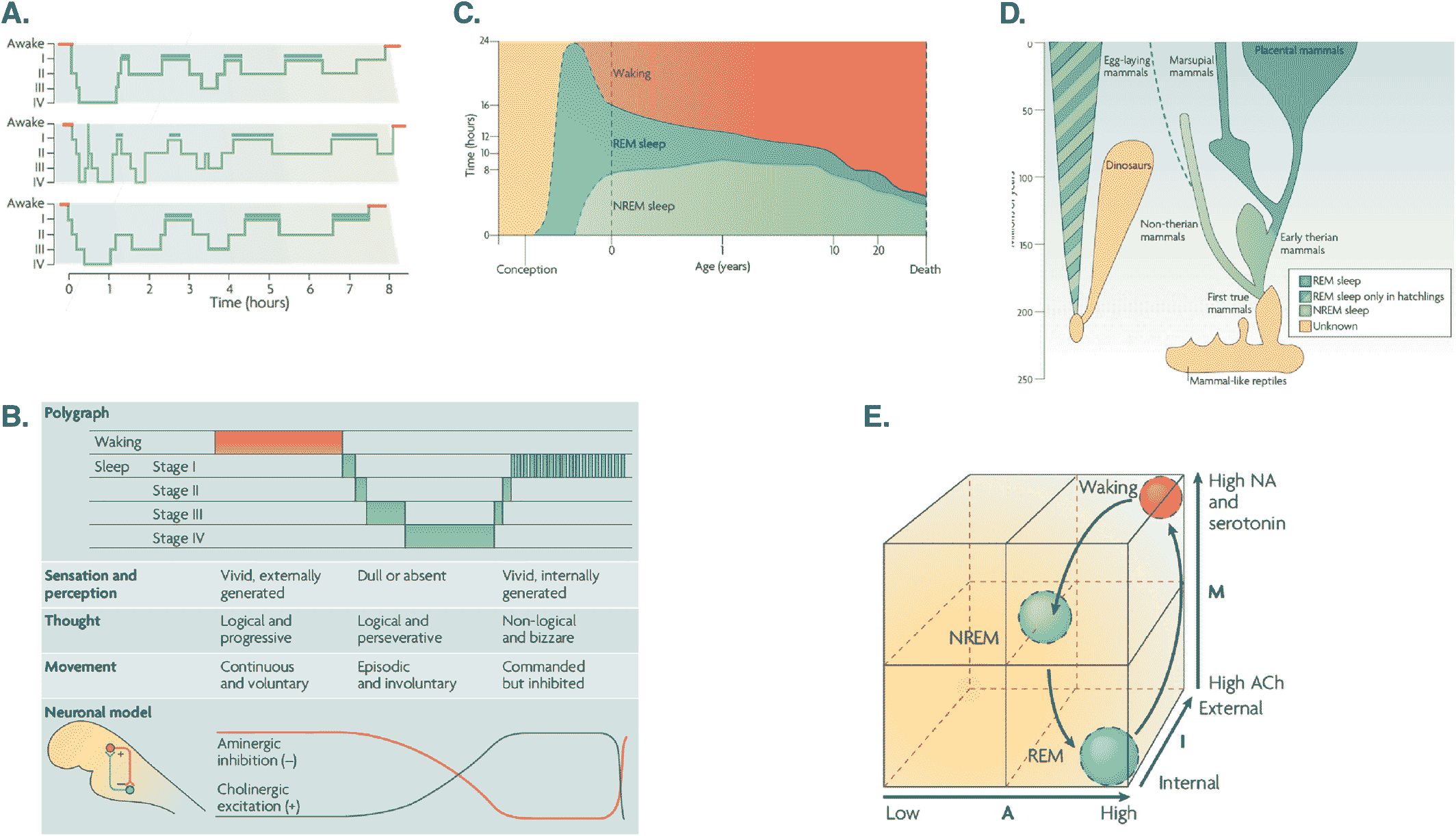
The AIM Model
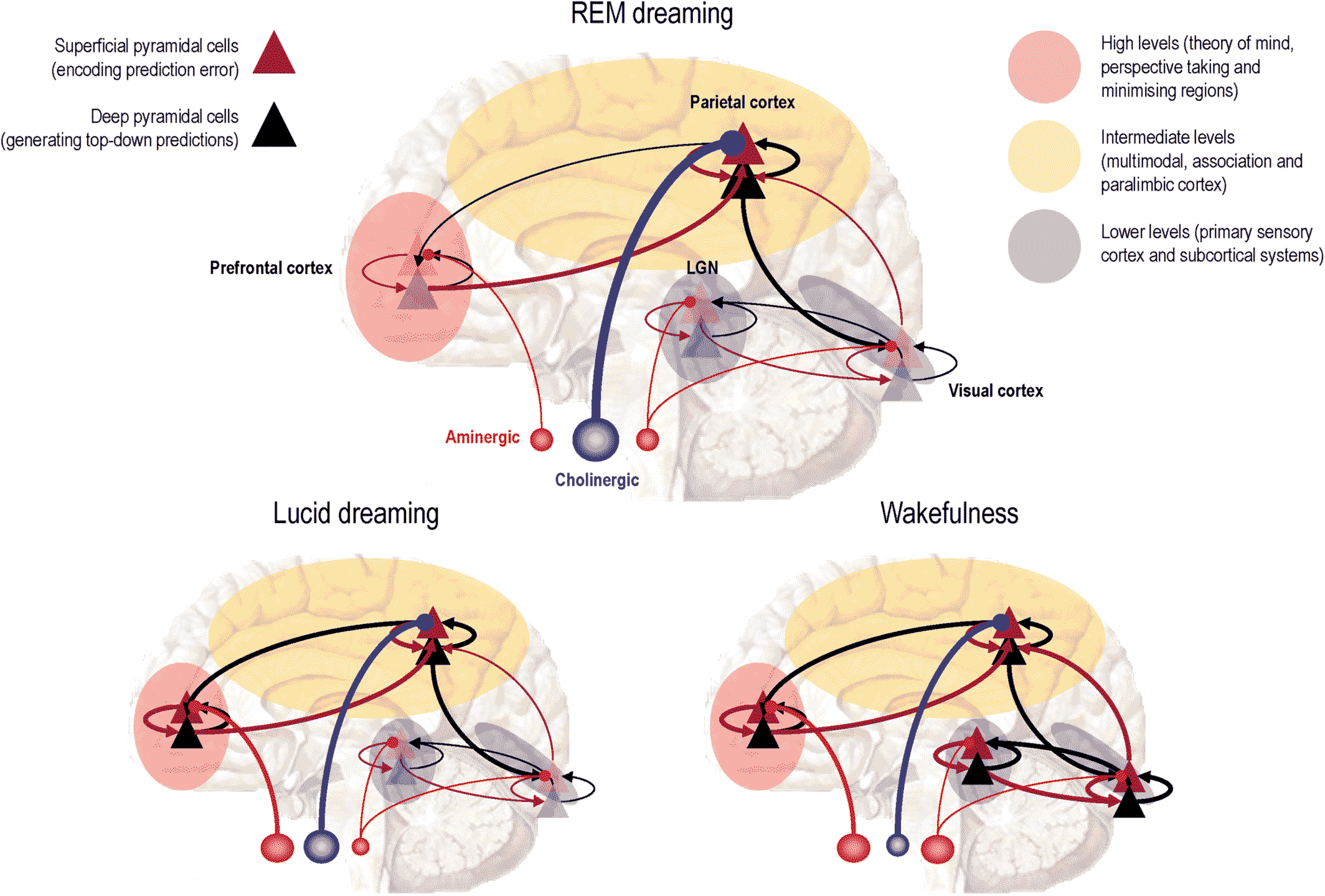
The Phantastic Organ
Precision Psychiatry
An Intuitive Physics for the Mind
Predictive Processing and Precision
Aberrant Precision
Syndrome symptom | Selected papers |
---|---|
Precision, predictive coding and Bayesian inference in schizorphenia | Pollak TA, Corlett PR. Blindness, psychosis, and the visual Construction of the world. Schizophr Bull. 2019 Oct 11. pii: sbz098. doi: https://doi.org/10.1093/schbul/sbz098. [Epub ahead of print] |
Benrimoh et al. (2019) (135) | |
Sterzer et al. (2018) (158) | |
Dzafic I, Burianová H, Martin AK, Mowry B. Neural correlates of dynamic emotion perception in schizophrenia and the influence of prior expectations. Schizophr Res. 2018 Dec; 202:129–137 | |
Heinz A, Murray GK, Schlagenhauf F, Sterzer P, Grace AA, Waltz JA. Towards a Unifying cognitive, neurophysiological, and computational neuroscience account of schizophrenia. Schizophr Bull. 2019 Sep 11; 45 (5):1092–1100 | |
Limongi R, Bohaterewicz B, Nowicka M, Plewka A, Friston KJ. Knowing when to stop: Aberrant precision and evidence accumulation in schizophrenia. Schizophr Res. 2018 Jul; 197:386–391 | |
Randeniya R, Oestreich LKL, Garrido MI. Sensory prediction errors in the continuum of psychosis. Schizophr Res. 2017 Apr 27. pii: S0920‐9964 (17)30206‐2 | |
Griffin JD, Fletcher PC. Predictive processing, source monitoring, and psychosis. Annu Rev Clin Psychol. 2017 May 8; 13:265–289 | |
Corlett PR. I predict, Therefore I Am: Perturbed predictive coding under ketamine and in schizophrenia. Biol Psychiatry. 2017 Mar 15; 81 (6):465–466 | |
Tschacher W, Giersch A, Friston K. Embodiment and schizophrenia: A review of implications and applications. Schizophr Bull. 2017 Mar 3 | |
van Schalkwyk GI, Volkmar FR, Corlett PR. A predictive coding account of psychotic symptoms in autism spectrum disorder. J Autism Dev Disord. 2017 May; 47 (5):1323–1340 | |
Schmack K, Rothkirch M, Priller J, Sterzer P. Enhanced predictive signalling in schizophrenia. Hum Brain Mapp. 2017 Apr; 38 (4):1767–1779 | |
Sterzer P, Mishara AL, Voss M, Heinz A. Thought Insertion as a self‐Disturbance: An integration of predictive coding and Phenomenological approaches. Front Hum Neurosci. 2016 Oct 12; 10:502. eCollection 2016 | |
Kort NS, Ford JM, Roach BJ, Gunduz‐Bruce H, Krystal JH, Jaeger J, Reinhart RM, Mathalon DH. Role of N‐Methyl‐D‐Aspartate receptors in action‐based predictive coding deficits in schizophrenia. Biol Psychiatry. 2017 Mar 15; 81 (6):514–524 | |
Friston K, Brown HR, Siemerkus J, Stephan KE. The dysconnection hypothesis (2016). Schizophr Res. 2016 Oct; 176 (2‐3):83–94 | |
Roa Romero Y, Keil J, Balz J, Gallinat J, Senkowski D. Reduced frontal theta oscillations indicate altered crossmodal prediction error processing in schizophrenia. J Neurophysiol. 2016 Sep 1; 116 (3):1396–1407 | |
Wacongne C. A predictive coding account of MMN reduction in schizophrenia. Biol Psychol. 2016 Apr; 116:68–74 | |
Powers et al. (2015) (136) | |
Adams RA, Huys QJ, Roiser JP. Computational psychiatry: Towards a mathematically informed understanding of mental illness. J Neurol Neurosurg Psychiatry. 2016 Jan; 87 (1):53–63 | |
Rentzsch J, Shen C, Jockers‐Scherübl MC, Gallinat J, Neuhaus AH. Auditory mismatch negativity and repetition suppression deficits in schizophrenia explained by irregular computation of prediction error. PLoS One. 2015 May 8; 10 (5):e0126775 | |
Castelnovo A, Ferrarelli F, D'Agostino A. Schizophrenia: From neurophysiological abnormalities to clinical symptoms. Front Psychol. 2015 Apr 20; 6:478 | |
Notredame et al. (2014) (137) | |
Fogelson et al. (2014) (149) | |
Horga G, Schatz KC, Abi‐Dargham A, Peterson BS. Deficits in predictive coding underlie hallucinations in schizophrenia. J Neurosci. 2014 Jun 11; 34 (24):8072–8082 | |
Jardri R, Denève S. Circular inferences in schizophrenia. Brain. 2013 Nov; 136(Pt 11):3227–3241 | |
Ford JM, Palzes VA, Roach BJ, Mathalon DH. Did I do that? Abnormal predictive processes in schizophrenia when button pressing to deliver a tone. Schizophr Bull. 2014 Jul; 40 (4):804–812 | |
Adams et al. (2013) (138) | |
Nazimek JM, Hunter MD, Woodruff PW. Auditory hallucinations: Expectation‐perception model. Med Hypotheses. 2012 Jun; 78 (6):802–810 | |
Lalanne L, van Assche M, Giersch A. When predictive mechanisms go wrong: Disordered visual synchrony thresholds in schizophrenia. Schizophr Bull. 2012 May; 38 (3):506–513 | |
Precision, predictive coding and Bayesian inference in autism and autistic spectrum disorder | Lanillos P, Oliva D, Philippsen A, Yamashita Y, Nagai Y, Cheng G. A review on neural network models of schizophrenia and autism spectrum disorder. Neural Netw. 2019 Nov 13; 122:338–363 |
Van de Cruys S, Perrykkad K, Hohwy J. Explaining hyper‐sensitivity and hypo‐responsivity in autism with a common predictive coding‐based mechanism. Cogn Neurosci. 2019 Jul; 10 (3):164–166 | |
Lawson et al. (2017) (133) | |
Chambon V, Farrer C, Pacherie E, Jacquet PO, Leboyer M, Zalla T. Reduced sensitivity to social priors during action prediction in adults with autism spectrum disorders. Cognition. 2017 Mar; 160:17–26 | |
van Schalkwyk GI, Volkmar FR, Corlett PR. A predictive coding account of psychotic symptoms in autism spectrum disorder. J Autism Dev Disord. 2017 May; 47 (5):1323–1340 | |
Van de Cruys S, Van der Hallen R, Wagemans J. Disentangling signal and noise in autism spectrum disorder. Brain Cogn. 2017 Mar; 112:78–83 | |
Manning C, Kilner J, Neil L, Karaminis T, Pellicano E. Children on the autism spectrum update their behaviour in response to a volatile environment. Dev Sci. 2016 Aug 6. doi: https://doi.org/10.1111/desc.12435 | |
Chan JS, Langer A, Kaiser J. Temporal integration of multisensory stimuli in autism spectrum disorder: a Predictive coding perspective. J Neural Transm (Vienna). 2016 Aug; 123 (8):917–923 | |
von der Lühe T, Manera V, Barisic I, Becchio C, Vogeley K, Schilbach L. Interpersonal predictive coding, not action perception, is impaired in autism. Philos Trans R Soc Lond B Biol Sci. 2016 May 5; 371 (1693) | |
Gonzalez‐Gadea ML, Chennu S, Bekinschtein TA, Rattazzi A, Beraudi A, Tripicchio P, Moyano B, Soffita Y, Steinberg L, Adolfi F, Sigman M, Marino J, Manes F, Ibanez A. Predictive coding in autism spectrum disorder and attention deficit hyperactivity disorder. J Neurophysiol. 2015 Nov; 114 (5):2625–2636 | |
Palmer CJ, Seth AK, Hohwy J. The felt presence of other minds: Predictive processing, counterfactual predictions, and mentalising in autism. Conscious Cogn. 2015 Nov; 36:376–389 | |
Precision, predictive coding and Bayesian inference in depression, stress and anxiety | Linson A, Parr T, Friston KJ. Active inference, stressors, and psychological trauma: A neuroethological model of (mal)adaptive explore‐exploit dynamics in ecological context. Behav Brain Res. 2019 Dec 9; 380:112421 |
Kube T, Schwarting R, Rozenkrantz L, Glombiewski JA, Rief W. Distorted cognitive processes in major depression: A predictive processing perspective. Biol Psychiatry. 2019 Jul 29. pii: S0006‐3223 (19)31550‐1 | |
Adams RA, Huys QJ, Roiser JP. Computational psychiatry: Towards a mathematically informed understanding of mental illness. J Neurol Neurosurg Psychiatry. 2016 Jan; 87 (1):53–63 | |
Clark et al. (2018) (128) | |
Adam Linson, Karl Friston. Reframing PTSD for computational psychiatry with the active inference framework. Cogn Neuropsychiatry. 2019; 24 (5): 347–368 | |
Barrett LF, Quigley KS, Hamilton P. An active inference theory of allostasis and interoception in depression. Philos Trans R Soc Lond B Biol Sci. 2016 Nov 19; 371 (1708). pii: 20160011 | |
Seth and Friston (2016) (97) | |
Stephan et al. (2016) (129) | |
Schutter DJ. A Cerebellar framework for predictive coding and Homeostatic Regulation in depressive disorder. Cerebellum. 2016 Feb; 15 (1):30–33 | |
Chekroud (2015) (168) | |
Cornwell et al. (2017) (127) | |
Kim MJ, Shin J, Taylor JM, Mattek AM, Chavez SJ, Whalen PJ. Intolerance of uncertainty predicts increased Striatal volume. Emotion. 2017 May 18 | |
Trapp S, Kotz SA. Predicting Affective information ‐ an evaluation of Repetition Suppression effects. Front Psychol. 2016 Sep 9; 7:1365 | |
Garfinkel SN, Seth AK, Barrett AB, Suzuki K, Critchley HD. Knowing your own heart: Distinguishing interoceptive accuracy from interoceptive awareness. Biol Psychol. 2015 Jan; 104:65–74 | |
Lawson RP, Rees G, Friston KJ. An aberrant precision account of autism. Front Hum Neurosci. 2014 May 14; 8:302 | |
Precision, predictive coding and Bayesian inference in hallucinations and hallucinosis | Powers AR, Corlett PR, Ross DA. Guided by Voices: Hallucinations and the psychosis spectrum. Biol Psychiatry. 2018 Sep 15; 84 (6):e43–e45 |
Powers et al. (2017) (64) | |
Corlett PR, Horga G, Fletcher PC, Alderson‐day B, Schmack K, powers AR 3rd. Hallucinations and Strong priors. Trends Cogn Sci. 2019 Feb; 23 (2):114–127 | |
Sterzer et al. (2018) (158) | |
O'Callaghan C, Hall JM, Tomassini A, Muller AJ, Walpola IC, Moustafa AA, Shine JM, Lewis SJG.Visual hallucinations are characterized by Impaired sensory evidence Accumulation: Insights from hierarchical Drift Diffusion modeling in Parkinson's disease. Biol Psychiatry Cogn Neurosci Neuroimaging. 2017 Nov; 2 (8):680–688 | |
Sterzer P, Mishara AL, Voss M, Heinz A. Thought Insertion as a self‐Disturbance: An integration of predictive coding and Phenomenological approaches. Front Hum Neurosci. 2016 Oct 12; 10:502 | |
Powers AR III, Kelley M, Corlett PR. Hallucinations as top‐down effects on perception. Biol Psychiatry Cogn Neurosci Neuroimaging. 2016 Sep; 1 (5):393–400 | |
Griffin JD, Fletcher PC. Predictive processing, source monitoring, and psychosis. Annu Rev Clin Psychol. 2017 May 8; 13:265–289 | |
Schmack K, Rothkirch M, Priller J, Sterzer P. Enhanced predictive signalling in schizophrenia. Hum Brain Mapp. 2017 Apr; 38 (4):1767–1779 | |
Sterzer P, Mishara AL, Voss M, Heinz A. Thought Insertion as a self‐Disturbance: An integration of predictive coding and Phenomenological approaches. Front Hum Neurosci. 2016 Oct 12; 10:502 | |
Roa Romero Y, Keil J, Balz J, Gallinat J, Senkowski D. Reduced frontal theta oscillations indicate altered crossmodal prediction error processing in schizophrenia. J Neurophysiol. 2016 Sep 1; 116 (3):1396–1407 | |
Teufel C, Subramaniam N, Dobler V, Perez J, Finnemann J, Mehta PR, Goodyer IM, Fletcher PC. Shift toward prior knowledge confers a perceptual advantage in early psychosis and psychosis‐prone healthy individuals. Proc Natl Acad Sci U S A. 2015 Oct 27; 112 (43):13401–13406 | |
Powers et al. (2015) (136) |
Summary
Discussion
Limitations
Footnotes
REFERENCES
Information & Authors
Information
Published In
History
Authors
Funding Information
Metrics & Citations
Metrics
Citations
Export Citations
If you have the appropriate software installed, you can download article citation data to the citation manager of your choice. Simply select your manager software from the list below and click Download.
For more information or tips please see 'Downloading to a citation manager' in the Help menu.
There are no citations for this item
View Options
View options
PDF/ePub
View PDF/ePubGet Access
Login options
Already a subscriber? Access your subscription through your login credentials or your institution for full access to this article.
Personal login Institutional Login Open Athens loginNot a subscriber?
PsychiatryOnline subscription options offer access to the DSM-5-TR® library, books, journals, CME, and patient resources. This all-in-one virtual library provides psychiatrists and mental health professionals with key resources for diagnosis, treatment, research, and professional development.
Need more help? PsychiatryOnline Customer Service may be reached by emailing [email protected] or by calling 800-368-5777 (in the U.S.) or 703-907-7322 (outside the U.S.).